Table of Contents
Abstract
This thesis examines the historical development, conceptual foundation, and future trajectory of Evidence-Based Medicine (EBM) as it evolves in an increasingly AI-driven healthcare landscape. By tracing EBM's journey from its philosophical origins to its current practice and emerging AI applications, this work demonstrates how EBM represents a fundamental epistemological shift in medicine—from authority-based to evidence-based knowledge. The research illuminates how this paradigm shift initially faced significant resistance before transforming into the dominant approach to clinical decision-making. As artificial intelligence increasingly augments human capabilities in evidence synthesis and application, EBM stands at the threshold of another transformation that may further enhance its impact while presenting new ethical and epistemological challenges. This thesis contributes to the understanding of how medical knowledge is created, evaluated, and applied, with implications for medical education, clinical practice, and healthcare policy in an AI-enhanced future.
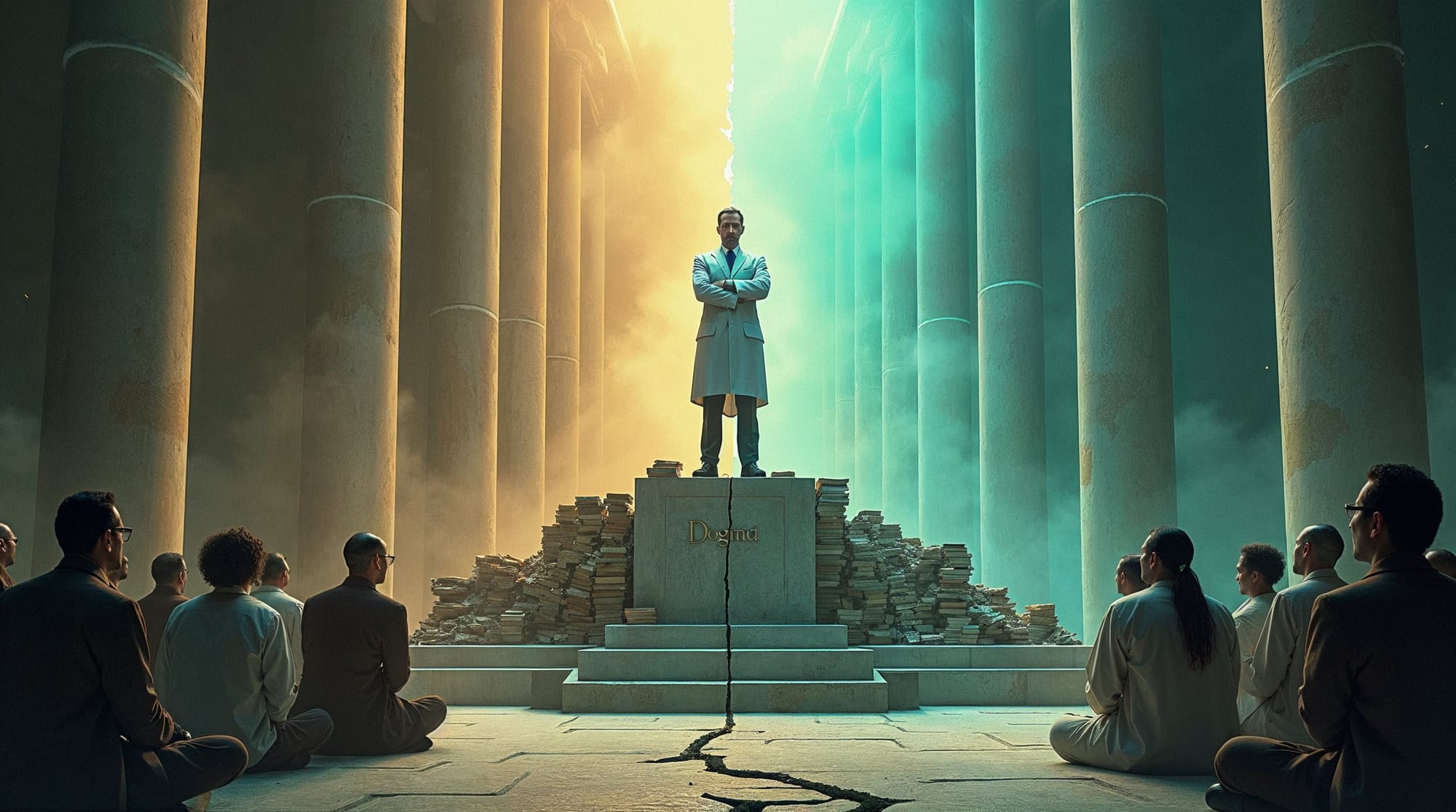
Chapter 1: Introduction and Background
1.1 The Central Problem: How Do We Know What Works?
At its core, medicine faces a fundamental question: How do we determine which interventions truly benefit patients? This seemingly simple inquiry has profound implications for patient outcomes, healthcare resources, and the very nature of medical practice. Throughout history, this question has been answered through various epistemological frameworks—from divine revelation and humoral theory to mechanistic reasoning and clinical experience. The development of Evidence-Based Medicine represents a distinct turn toward empirical verification as the primary basis for clinical decisions.
1.2 Definition and Scope of Evidence-Based Medicine
Evidence-Based Medicine emerged formally in the early 1990s, with David Sackett providing what became its definitive definition: "the conscientious, explicit, and judicious use of current best evidence in making decisions about the care of individual patients." This definition encompasses three crucial elements that distinguish EBM from previous approaches: the systematic search for and appraisal of evidence, the integration of this evidence with clinical expertise, and the incorporation of patient values and preferences. While seemingly straightforward, this definition represents a radical departure from traditional medical practice that prioritized authority, experience, and pathophysiological reasoning over empirical evidence.
1.3 Thesis Structure and Methodology
This thesis employs historical analysis, conceptual exploration, and forward-looking research to examine EBM's evolution. It traces the movement from its philosophical roots through its formalization, initial resistance, eventual acceptance, and current integration with artificial intelligence technologies. Through this multifaceted exploration, the thesis aims to illuminate not only what EBM is but why it emerged, how it transformed medical practice, and where it might be headed in an AI-enhanced future. This comprehensive approach reveals EBM not merely as a method for clinical decision-making but as a fundamental epistemological framework that continues to evolve as technology transforms the generation, synthesis, and application of medical knowledge.
Chapter 2: Historical Origins of Evidence-Based Medicine
2.1 Precursors to EBM: The Quest for Empirical Medicine
While Evidence-Based Medicine as a named movement began in the early 1990s, its philosophical foundations extend much further back. The quest for empirical validation in medicine can be traced to mid-19th century Paris, where Pierre Louis conducted some of the earliest clinical epidemiology work. Louis's "numerical method" challenged established treatments like bloodletting by systematically collecting and analyzing patient outcomes, representing an early form of comparative effectiveness research.
Several key developments in the 20th century laid the groundwork for EBM's eventual emergence:
- John R. Paul coined the term "clinical epidemiology" in 1938 while working at Yale School of Medicine
- Alvan R. Feinstein's work at Yale in the 1960s introduced rigorous statistical methods to quantify clinical practices
- Archie Cochrane's influential 1972 book "Effectiveness and Efficiency: Random Reflections on Health Services" highlighted the lack of evidence behind many medical practices and advocated for randomized controlled trials
These forerunners began the shift from practice based primarily on authority and tradition toward one grounded in empirical evidence.
2.2 Medical Practice Before EBM: The Authority-Based Paradigm
Before EBM's emergence, medical practice operated under fundamentally different principles:
Medical decisions were typically based on the authority and experience of senior physicians, with knowledge passed down through an apprenticeship model. The opinions of experts were rarely questioned, and clinical reasoning relied heavily on pathophysiological theory rather than direct evidence of patient outcomes. Medicine was dominated by simple "if-then" rules presented without evidence beyond being "standard practice," and the "art of medicine" was emphasized over scientific evidence.
This approach led to widespread variations in medical practice, the continued use of ineffective or harmful treatments, and slow adoption of beneficial innovations. The gap between biomedical research and clinical practice remained wide, with many research findings taking decades to influence routine care—if they ever did at all.
2.3 Key Historical Figures in EBM Development
2.3.1 David Sackett: The Father of Evidence-Based Medicine
David Sackett (1934-2015) stands as the most influential figure in EBM's development. Born in Chicago and trained at the University of Illinois and Harvard, Sackett founded the first Department of Clinical Epidemiology and Biostatistics at McMaster University in 1967—the first academic unit of its kind worldwide.
Sackett's contributions were transformative. He pioneered methods for critical appraisal of medical literature and developed approaches for applying research findings to clinical practice. His philosophical approach emphasized the integration of clinical expertise with external evidence while respecting patient values—a balanced perspective that would define EBM's mature form.
In 1996, Sackett co-authored the seminal paper "Evidence based medicine: what it is and what it isn't" in the British Medical Journal, which provided EBM's definitive definition and addressed many early misconceptions. After leaving McMaster, he founded the Oxford Centre for Evidence-Based Medicine, further institutionalizing the movement. His textbooks, including "Clinical Epidemiology: A Basic Science for Clinical Medicine" and "Evidence-Based Medicine: How to Practice and Teach EBM," became foundational texts that shaped how generations of clinicians approached medical evidence.
2.3.2 Archie Cochrane: The Intellectual Predecessor
Archibald "Archie" Cochrane (1909-1988) laid crucial intellectual foundations for EBM decades before the term was coined. Born in Scotland and trained at Cambridge and London, Cochrane's experiences as a prisoner of war during World War II—where he witnessed the effects of untested medical interventions—profoundly shaped his commitment to scientific evidence in healthcare.
His 1972 book "Effectiveness and Efficiency: Random Reflections on Health Services" strongly advocated for randomized controlled trials to guide clinical practice. He famously called for "a critical summary, by specialty, of all relevant randomized controlled trials"—a vision that would later inspire the Cochrane Collaboration. Cochrane's passionate advocacy for using scientific evidence to evaluate healthcare interventions earned him the status of intellectual godfather to the EBM movement.
2.3.3 Iain Chalmers: Architect of Systematic Review
Sir Iain Chalmers (b. 1943) translated Cochrane's vision into a practical reality through the creation of the Cochrane Collaboration. After qualifying in medicine in the 1960s and practicing in the UK and Gaza Strip, Chalmers became the first director of the National Perinatal Epidemiology Unit in Oxford from 1978 to 1992.
There, he led the development of the Oxford Database of Perinatal Trials and co-authored the landmark "Effective Care in Pregnancy and Childbirth," considered the first truly evidence-based textbook. In 1992, he was appointed director of the UK Cochrane Centre, leading to the development of the international Cochrane Collaboration in 1993.
Chalmers championed the systematic collection and synthesis of evidence through systematic reviews and meta-analyses. As founding editor of the James Lind Library, which documents the evolution of fair trials of treatments, he connected modern EBM to its historical roots. His work establishing the Cochrane Collaboration created an enduring infrastructure for evidence synthesis that remains central to EBM practice today.
2.3.4 Gordon Guyatt: The Name-Giver
Gordon Guyatt (b. 1953), a Canadian physician trained at McMaster University under David Sackett, coined the term "evidence-based medicine" in 1991 in an editorial for the ACP Journal Club. This followed his earlier attempt to call the approach "scientific medicine," which had met resistance from colleagues who felt it implied their existing practices were unscientific.
Guyatt led the Evidence-Based Medicine Working Group that published the landmark 1992 JAMA article introducing EBM to the broader medical community. As Program Director of Internal Medicine at McMaster from 1990 to 1997, he implemented the first evidence-based curriculum in medical education.
His most enduring methodological contribution may be leading the development of the GRADE (Grading of Recommendations Assessment, Development and Evaluation) approach for evaluating evidence quality, which has become the most widely adopted system for evidence appraisal worldwide.
2.4 The Formal Birth of EBM
The formal birth of Evidence-Based Medicine as a named movement occurred in the early 1990s at McMaster University, where a critical mass of clinical epidemiologists had gathered under Sackett's leadership. The timeline of its emergence reveals a rapid evolution:
- 1990: Gordon Guyatt first uses the term "Scientific Medicine" at McMaster, later changing it to "Evidence-Based Medicine"
- 1991: Guyatt publishes an editorial in ACP Journal Club using the term "evidence-based medicine"
- 1992: The Evidence-Based Medicine Working Group publishes "Evidence-based medicine: A new approach to teaching the practice of medicine" in JAMA
- 1993: The Cochrane Collaboration is established, named in honor of Archie Cochrane
- 1995: Launch of the journal "Evidence-Based Medicine"
- 1996: Sackett and colleagues publish the definitive definition of EBM in BMJ
This rapid sequence of developments in the early 1990s transformed what had been a relatively obscure approach at McMaster into an international movement that would eventually reshape medical education, research, and practice worldwide.
Chapter 3: The Conceptual Framework and Methodology of EBM
3.1 The Three Pillars of EBM
The conceptual foundation of Evidence-Based Medicine rests on three interdependent pillars:
Best Research Evidence forms the first pillar, encompassing high-quality, clinically relevant research from patient-centered clinical studies. This includes evidence about the accuracy of diagnostic tests, the power of prognostic markers, and the efficacy and safety of therapeutic, rehabilitative, and preventive interventions. The quality and strength of this evidence exist along a continuum, with well-designed studies providing stronger foundations for clinical decisions.
Clinical Expertise constitutes the second pillar, acknowledging the proficiency and judgment that individual clinicians acquire through clinical experience and practice. This expertise enables more effective diagnosis and the thoughtful identification of individual patients' situations, rights, and preferences. Contrary to early mischaracterizations of EBM, clinical expertise remains central to the approach, serving as the means to integrate evidence with the unique characteristics of each patient.
Patient Values and Preferences form the third pillar, recognizing the unique preferences, concerns, and expectations that patients bring to the clinical encounter. These values must be integrated into clinical decisions if the evidence is to serve individual patients effectively. The incorporation of patient values represents the recognition that medical decisions are not merely technical but inherently value-laden.
These three pillars were not always equally emphasized in early EBM descriptions, with later formulations giving greater prominence to patient values as the movement matured. When properly balanced, these components enable clinical decisions that are scientifically sound, clinically nuanced, and aligned with patient priorities.
3.2 The Five-Step EBM Process
EBM is implemented through a systematic five-step process that transforms the approach from abstract principle to practical methodology:
- Ask: Converting the need for information into an answerable clinical question, typically using the PICO framework (Patient/Problem, Intervention, Comparison, Outcome) to structure inquiries. This critical first step transforms vague clinical uncertainties into questions that can be systematically investigated.
- Acquire: Tracking down the best evidence to answer the question, using efficient search strategies that prioritize pre-appraised resources when available. This step has been transformed by digital resources that make evidence far more accessible than in EBM's early days.
- Appraise: Critically evaluating the evidence for its validity, impact, and applicability. This step employs systematic approaches to assess methodological quality, magnitude of effects, and relevance to specific patient populations.
- Apply: Integrating the evidence with clinical expertise and patient values to make a clinical decision. This integration represents the art of medicine within the scientific framework of EBM.
- Assess: Evaluating the effectiveness and efficiency of the process and seeking ways to improve. This self-reflective component makes EBM a continuously evolving approach rather than a static methodology.
This process replaced the traditional approach of consulting authorities or textbooks with a more rigorous method that enables clinicians to find, evaluate, and apply the most current evidence to individual patient care.
3.3 The Hierarchy of Evidence
A cornerstone of EBM methodology is the recognition that not all evidence is created equal. The hierarchy of evidence ranks study designs according to their potential for bias and methodological rigor:
At the apex of traditional evidence hierarchies sit systematic reviews and meta-analyses, which provide comprehensive syntheses of available evidence with methods designed to minimize bias. These are followed by randomized controlled trials (RCTs), which minimize selection bias and control for confounding factors through random allocation of participants.
Lower in the hierarchy are observational studies including cohort studies (following groups over time) and case-control studies (comparing those with and without an outcome), which provide valuable evidence particularly when RCTs are impractical or unethical. At the base of the pyramid are case series, case reports, and expert opinion, which offer the weakest form of evidence due to their high potential for bias.
This hierarchy has evolved significantly since its introduction. The Oxford Centre for Evidence-Based Medicine Levels of Evidence, initially developed in 1998 and revised in 2011, tailors evidence hierarchies to different types of clinical questions. The GRADE approach, developed in the early 2000s, provides a more nuanced framework by considering not just study design but also risk of bias, inconsistency, indirectness, imprecision, and publication bias.
These refinements acknowledge that the quality of evidence depends not only on study design but also on methodological quality, consistency across studies, and applicability to clinical practice—moving beyond the early, more rigid hierarchies that sometimes oversimplified the complex nature of evidence.
3.4 Critical Appraisal: The Heart of EBM
Critical appraisal—the systematic evaluation of research to assess its validity, results, and relevance—represents the intellectual core of EBM. This practice transformed clinicians from passive consumers of medical literature to active evaluators capable of distinguishing strong evidence from weak.
Critical appraisal involves systematically evaluating three key aspects of research:
- Validity: Was the study well-designed and executed with minimal bias?
- Importance: What is the magnitude and precision of the effects?
- Applicability: Can the results be applied to a specific patient or population?
Several tools facilitate this process, including the Users' Guides to the Medical Literature, Critical Appraisal Skills Programme (CASP) checklists, and specialty-specific instruments. These tools provide structured frameworks for evaluating different study designs, from randomized trials to qualitative research.
The development of critical appraisal skills marked a significant shift in medical education, moving from memorization of facts to the development of lifelong learning skills that enable clinicians to continuously update their knowledge base as evidence evolves.
Chapter 4: From Resistance to Revolution: EBM's Path to Acceptance
4.1 Initial Skepticism and Resistance to EBM
The introduction of Evidence-Based Medicine in the early 1990s faced substantial resistance from various segments of the medical community, revealing deep tensions in how medical knowledge is conceptualized and valued.
Philosophical and epistemological objections formed one category of resistance. Many physicians held that medicine was fundamentally an "art" that couldn't be reduced to statistical formulas or algorithms. Critics argued that EBM's emphasis on randomized controlled trials neglected other valuable forms of knowledge, including clinical experience and pathophysiological reasoning. Some saw EBM as reductionist, failing to account for the complexity and individuality of patient care.
Practical concerns constituted another source of resistance. Implementing EBM required new skills that many practicing physicians lacked, including literature searching and critical appraisal. Time constraints in busy clinical practices made it difficult for physicians to search for and evaluate evidence for every clinical question. Limited access to evidence resources, especially in the pre-internet era, posed significant practical barriers.
Professional identity and autonomy concerns represented perhaps the most emotionally charged objections. Many physicians viewed EBM as a threat to their professional autonomy and clinical judgment. The term "cookbook medicine" emerged as a pejorative, suggesting that EBM would reduce the physician's role to merely following algorithms. There was concern that EBM would be used by administrators and payers to control physician behavior and limit treatment options.
Traditional medical educators argued that EBM devalued clinical experience and the mentor-apprentice model of medical education. Specialty societies initially resisted evidence-based guidelines that contradicted established practice patterns. Philosophers of medicine questioned the epistemological foundations of EBM, arguing that its hierarchy of evidence was arbitrary and privileged certain forms of knowledge over others.
4.2 The Gradual Process of Acceptance
Several factors facilitated the increasing acceptance of EBM over time, transforming it from a controversial approach to the dominant paradigm in medicine.
Technological and information advances played a crucial role. The rise of the internet and digital databases made accessing medical literature much easier. Development of tools like PubMed, the Cochrane Database of Systematic Reviews, and clinical decision support systems made practicing EBM more feasible. New journals dedicated to EBM and systematic reviews provided readily accessible evidence summaries.
Institutional support provided structural momentum. The formation of the Cochrane Collaboration in 1993 provided a framework for systematic reviews. Government agencies like the Agency for Healthcare Research and Quality (AHRQ) in the US and the National Institute for Health and Care Excellence (NICE) in the UK endorsed and promoted evidence-based approaches. Medical schools began incorporating EBM into their curricula, creating a new generation of physicians trained in EBM principles.
Perhaps most importantly, EBM itself evolved in response to criticisms. Proponents responded by emphasizing that evidence should complement, not replace, clinical expertise and patient values. Sackett and colleagues refined the definition of EBM to explicitly include clinical expertise and patient preferences alongside research evidence. The development of more nuanced evidence hierarchies and grading systems acknowledged the value of different types of evidence for different questions.
The pace and extent of EBM adoption varied considerably across different medical specialties. Internal medicine, the birthplace of EBM, remained at the forefront of its adoption. Cardiology embraced EBM relatively early, with large RCTs becoming the foundation for practice guidelines. Obstetrics and gynecology saw widespread adoption of evidence-based guidelines, particularly following criticisms that many common practices lacked evidence of effectiveness.
Other specialties adopted EBM more gradually. Pediatrics developed a strong EBM tradition but faced challenges due to ethical constraints on pediatric research. Psychiatry initially showed resistance but eventually developed evidence-based treatment guidelines. Surgery was initially resistant due to challenges in conducting surgical RCTs but gradually embraced EBM principles with adaptations for surgical practice.
4.3 Institutionalization in Medical Education and Practice
By the early 2000s, EBM had become firmly institutionalized in medical education worldwide, marking its transition from innovative approach to standard practice.
Medical schools in Canada, the US, UK, Australia, and other countries had incorporated EBM into their curricula through various approaches:
- Dedicated EBM courses, often placed strategically after initial clinical experiences
- Integration of EBM principles throughout the curriculum
- Journal clubs and critical appraisal exercises
- Problem-based learning incorporating EBM skills
- Clinical clerkships with EBM components
Residency programs across specialties developed EBM curricula featuring regular journal clubs, critical appraisal exercises, and evidence-based case presentations. Professional organizations developed EBM resources and continuing education focused on evidence-based practice. Board certification and recertification requirements increasingly incorporated EBM knowledge and skills.
A vast infrastructure developed to support EBM practice, including:
- Information resources like the Cochrane Database of Systematic Reviews and point-of-care tools
- Institutional structures such as Evidence-Based Practice Centers established by AHRQ
- Methodological advances including standardized reporting guidelines and systems for rating evidence quality
By 2007, when the British Medical Journal conducted an online poll ranking EBM seventh among the 15 most important milestones in modern medicine, the transformation was complete. What had begun as a radical challenge to medical authority had become the new authority—a dominant paradigm that would itself face new challenges and evolution in the coming decades.
4.4 How EBM Transformed Medicine
EBM fundamentally altered how clinical decisions are made, with the decision-making framework shifting from reliance on authority, tradition, and unsystematic observations to the integration of clinical expertise, patient values, and best available evidence. Clinical questions became more structured, often following the PICO format. Physicians became more critical consumers of medical literature, questioning established practices that lacked evidence.
The impact on healthcare quality has been measurable, with many practices previously based solely on tradition or expert opinion replaced by evidence-supported interventions. The development and implementation of evidence-based guidelines has standardized care for many conditions, reducing unwarranted variation. High-quality systematic reviews have identified both beneficial treatments that were underused and ineffective or harmful interventions that were overused.
EBM extended beyond individual clinical decisions to influence health systems and policy. Health technology assessment using evidence-based methods became standard for decisions about coverage and reimbursement. Pay-for-performance and quality improvement initiatives increasingly focused on evidence-based measures. Evidence-based guidelines became central to defining standards of care, with legal and regulatory implications.
The case of hormone replacement therapy (HRT) illustrates how EBM transformed medical practice. For decades, HRT was routinely prescribed for postmenopausal women based on pathophysiological reasoning and observational studies suggesting cardiovascular benefits. In 2002, the Women's Health Initiative, a large RCT, found that combined estrogen-progestin HRT actually increased the risk of breast cancer, heart disease, stroke, and blood clots. This evidence rapidly transformed clinical practice, with HRT prescriptions dropping significantly and prescribing patterns becoming more selective. The case demonstrated how evidence could overturn established practices based on traditional thinking, even when those practices were widely accepted by medical authorities.
Chapter 5: The Current State of Evidence-Based Medicine
5.1 Current Implementation Across Medical Specialties
Evidence-Based Medicine has become the dominant paradigm across medical specialties, though implementation varies considerably based on specialty characteristics and available evidence.
In primary care, physicians apply EBM through clinical practice guidelines, point-of-care tools, and decision support systems. However, time constraints and the broad range of conditions encountered often challenge thorough evidence review. The emphasis on preventive care in primary care has led to particularly strong evidence-based approaches to screening and health maintenance.
Specialty medicine fields like cardiology and oncology have extensively adopted EBM, with robust clinical guidelines and pathways based on high-quality evidence from randomized controlled trials. These specialties benefit from substantial research funding that supports large-scale trials and sophisticated meta-analyses to guide practice.
Surgical specialties have had a more complex relationship with EBM, as randomized controlled trials are sometimes difficult to conduct for surgical interventions. Alternative study designs and outcomes measures have been developed to address these challenges. Despite initial resistance, fields like orthopedics and vascular surgery have developed substantial evidence bases to guide procedural decisions.
Mental health disciplines have incorporated EBM into practice guidelines while acknowledging the challenges in standardizing interventions and measuring outcomes. The development of manualized therapies and standardized outcome measures has facilitated more evidence-based approaches to psychological treatments.
Emergency medicine has adapted EBM to high-acuity, time-sensitive situations through the development of clinical decision rules and rapid protocols based on best available evidence. The specialty has embraced pragmatic trials that reflect the realities of emergency care.
5.2 Challenges and Criticisms of Current EBM Methodology
Despite its widespread adoption, contemporary EBM faces significant methodological challenges that limit its effectiveness and application.
Evidence gaps persist for many clinical questions, particularly for rare diseases, pediatric populations, pregnant women, and older adults with multiple comorbidities. When high-quality evidence doesn't exist, clinicians must rely on lower-quality evidence or clinical judgment, creating tensions with EBM's emphasis on higher-level evidence.
Generalizability issues remain problematic, as evidence from tightly controlled trials may not transfer well to diverse real-world populations. Most clinical trials exclude patients with multiple comorbidities, leading to an "evidence-comorbidity gap" where the patients most commonly seen in practice are least represented in research.
Publication bias—the tendency to publish positive rather than negative findings—continues to distort the evidence base, overestimating treatment benefits. Despite efforts like trial registration requirements, selective outcome reporting and non-publication of negative results remain common.
Practical implementation barriers include time constraints, information overload, and skills gaps. Clinicians often lack sufficient time to search for, appraise, and apply research evidence during busy clinical practice. The volume of medical research publications continues to grow exponentially, making it impossible for practitioners to keep up without specialized tools.
Philosophical and conceptual criticisms challenge EBM's foundations. Some critics argue that EBM's focus on quantitative research methods reduces complex clinical scenarios to oversimplified statistical models. The hierarchy that places RCTs at the top may devalue other important forms of evidence, including qualitative research and clinical expertise.
Health disparities and equity issues represent growing concerns. Clinical trials often underrepresent important populations including women, minorities, elderly, and those with multiple conditions. Access to evidence-based care varies significantly across socioeconomic divides, geographic locations, and healthcare systems.
5.3 Current Tools and Technologies Supporting EBM Practice
Modern EBM implementation relies on sophisticated tools and technologies that have evolved significantly since the movement's early days.
Electronic clinical decision support systems have enhanced EBM implementation through point-of-care tools like UpToDate, DynaMed, and BMJ Best Practice that provide clinicians with rapid access to evidence summaries. EHR-integrated decision support offers evidence-based alerts, order sets, and care pathways within electronic health records. Mobile applications provide immediate access to evidence resources at the bedside.
Evidence repositories and databases support evidence-based practice through collections like the Cochrane Library, the premier source of systematic reviews. Guidelines repositories from organizations like the National Guideline Clearinghouse and NICE provide ready access to evidence-based recommendations. Primary literature databases including PubMed, EMBASE, and CINAHL facilitate access to original research reports.
Knowledge translation tools facilitate evidence implementation through frameworks like the Knowledge to Action model that guide evidence implementation in clinical settings. Decision aids help patients and clinicians make shared decisions based on evidence and patient preferences. Quality improvement methodologies like Plan-Do-Study-Act cycles structure the implementation of evidence in clinical systems.
5.4 Early Integration of AI and Computational Methods
Artificial intelligence and computational methods are beginning to transform evidence synthesis and application, addressing some of EBM's most persistent challenges.
Machine learning for literature screening has shown promising results in systematic review processes. Tools like Abstrackr, RobotReviewer, and ASReview use machine learning to screen titles and abstracts, reducing workload by 30-70%. Citation screening automation identifies potentially relevant citations based on previous screening decisions, accelerating the review process.
Natural language processing in evidence extraction enables automated data extraction from research reports, identifying key information about study characteristics, populations, interventions, and outcomes. Systems like RobotReviewer automatically evaluate methodological quality and potential biases in research studies. Text mining applications analyze large textual datasets to identify patterns, relationships, and evidence clusters.
The Cochrane Collaboration has pioneered several automation initiatives, including Project Transform, which uses machine learning to screen thousands of trial reports and identify those most likely relevant for Cochrane Reviews. Living Systematic Reviews maintained by Cochrane use automation to incorporate new evidence as it emerges, addressing the problem of reviews becoming quickly outdated.
These early applications of AI in evidence synthesis demonstrate the potential for technology to address some of EBM's most persistent challenges, particularly the volume-velocity problem of keeping up with rapidly expanding medical literature.
Chapter 6: The Future of EBM in an AI Reality
6.1 AI Technologies Transforming Evidence Synthesis and Appraisal
Artificial intelligence technologies are poised to fundamentally transform how medical evidence is synthesized, appraised, and applied, addressing many of the limitations that have constrained EBM's effectiveness.
Advanced natural language processing and large language models are revolutionizing evidence synthesis. Tools like GPT-4 and Claude are being explored for generating research summaries, formulating research questions, and drafting sections of systematic reviews. Semantic analysis capabilities enable understanding of meaning and context in medical literature, not just keyword matching. Multilingual NLP expands the scope of evidence available for synthesis beyond English-language sources.
AI for evidence appraisal and quality assessment is increasingly sophisticated. Automated critical appraisal systems assess the methodological quality of studies using recognized frameworks like GRADE, potentially improving the consistency and efficiency of quality assessment. Bias detection algorithms identify potential biases in research, including selection bias, reporting bias, and conflicts of interest. Inconsistency identification systems detect contradictions across studies and evaluate the coherence of the evidence base.
Continuous evidence monitoring systems are emerging that can track the medical literature in real-time, alerting researchers and clinicians when new evidence relevant to specific questions becomes available. These systems could transform the currently episodic nature of evidence synthesis into a continuous process that keeps pace with rapidly evolving research.
6.2 From Periodic to Continuous Evidence
Perhaps the most fundamental paradigm shift that AI enables is the transition from episodic to continuous evidence synthesis and application.
Living systematic reviews supported by AI tools like TrialMind and the Living Interactive Evidence Synthesis Framework enable "living" reviews that update automatically as new evidence emerges. The Norwegian Institute of Public Health's implementation study demonstrates significant efficiency gains from machine learning in evidence synthesis workflows, reducing time-to-completion by 60-80%.
Automated evidence processing systems can screen thousands of publications, extract relevant data, and synthesize findings with minimal human intervention. Cochrane's machine learning technologies identify relevant studies with high recall rates (0.897-1.000) when screening from over 20 million PubMed studies, dramatically accelerating the evidence synthesis process.
Integration of diverse evidence types beyond randomized controlled trials, including real-world data, patient-reported outcomes, and observational studies, is increasingly feasible with AI methods. These systems can systematically integrate diverse data sources to provide a more comprehensive evidence base, potentially addressing one of the persistent criticisms of traditional EBM.
6.3 The Shift from Population to Precision Evidence
AI technologies are enabling a fundamental shift from population-based to precision evidence, addressing one of the core tensions in traditional EBM.
Precision evidence matching algorithms match patients with the most relevant evidence based on their specific characteristics, conditions, and contexts, moving beyond the traditional one-size-fits-all approach of population-based evidence. This approach acknowledges that average treatment effects may not apply equally to all individuals.
Individualized treatment effect prediction combines advanced causal inference techniques with machine learning to better predict how individual patients will respond to treatments, rather than relying solely on average effects across populations. These methods can incorporate genetic, physiological, environmental, and behavioral factors to create truly personalized evidence.
Contextual evidence relevance assessment uses AI to evaluate the applicability of evidence to specific contexts—particular patient populations, healthcare settings, resource constraints—rather than assuming universal relevance. This approach recognizes the importance of implementation context in determining whether evidence-based interventions will achieve their expected benefits.
6.4 Future Presentation of Evidence
How evidence is presented to clinicians and patients will be transformed through AI-enabled approaches that make complex information more accessible and actionable.
Conversational evidence interfaces like ClinicalKey AI demonstrate how clinicians can access evidence through natural language queries rather than structured database searches, making evidence more accessible in clinical workflows. Context-aware evidence summaries adapt to the specific clinical scenario, highlighting the most relevant information based on the patient's condition and treatment history. Dialogue-based evidence exploration allows clinicians to interrogate the evidence, asking follow-up questions and probing uncertainties interactively.
Visual and interactive evidence presentation will make complex evidence more comprehensible. Dynamic evidence dashboards will allow users to explore evidence at different levels of detail, from high-level summaries to granular analysis of specific studies. Advanced techniques for visualizing uncertainty will better represent confidence intervals and limitations in the evidence, helping clinicians understand where knowledge is solid and where it remains uncertain. Personalized visualizations will adapt to individual clinician preferences and cognitive styles, recognizing that different users process information differently.
Multi-modal evidence integration will synthesize findings from different sources (clinical trials, observational studies, patient-reported outcomes) into cohesive presentations that highlight complementary insights. Temporal views will show how evidence has evolved over time, enabling clinicians to understand the trajectory of knowledge in a field.
6.5 Ethical Considerations in AI-Enhanced EBM
The integration of AI into evidence-based medicine raises significant ethical considerations that must be addressed for these technologies to enhance rather than undermine the goals of EBM.
Data privacy and consent issues become increasingly complex as AI systems process vast amounts of healthcare data. Traditional models of informed consent may be insufficient for AI systems that continuously analyze data and generate new insights. Robust governance frameworks and transparent data usage policies will be essential to maintain trust in AI-enhanced evidence systems.
Algorithmic bias and health equity concerns are paramount, as AI systems risk perpetuating or amplifying existing biases in healthcare. If these systems are trained on biased datasets (e.g., underrepresenting certain populations), the resulting evidence may be less applicable to underrepresented groups. Ensuring that AI-enhanced evidence benefits all populations equally will require intentional design and continuous monitoring for disparate impacts.
Transparency and explainability represent particular challenges, as the "black box" nature of many AI systems threatens the transparency fundamental to EBM. Future AI systems will need to explain their reasoning and the evidence upon which recommendations are based. The processes by which AI systems synthesize and apply evidence must be auditable to ensure methodological rigor.
The changing role of clinical judgment must be carefully considered as AI systems become more sophisticated. Rather than replacing clinical judgment, AI should complement it, with humans and machines each contributing their unique strengths to evidence-based decision-making. Clinicians will need metacognitive skills to determine when to rely on AI recommendations and when to override them based on contextual factors not captured in the algorithms.
Chapter 7: Conclusion and Implications
7.1 EBM's Enduring Legacy and Continuing Evolution
Evidence-Based Medicine represents one of the most significant epistemological shifts in the history of medicine—a transformation in how medical knowledge is created, evaluated, and applied. From its philosophical roots in mid-19th century empiricism to its formal articulation in the 1990s and its current integration with artificial intelligence, EBM has continuously evolved while maintaining its core commitment to grounding clinical decisions in rigorous evidence.
The movement's fundamental contribution lies in its systematic approach to evaluating medical knowledge, replacing authority and tradition with empirical verification as the primary basis for clinical decisions. By developing methodologies for critically appraising evidence and frameworks for applying that evidence in clinical practice, EBM has equipped generations of clinicians with tools to navigate an increasingly complex medical literature.
The key figures in EBM's development—Sackett, Cochrane, Chalmers, Guyatt, and others—demonstrated remarkable vision in identifying the limitations of traditional medical practice and crafting a more rigorous alternative. Their intellectual and institutional contributions created a foundation that continues to support evidence-based practice worldwide.
As EBM continues to evolve in an AI-enhanced world, it faces both new opportunities and challenges. Artificial intelligence promises to address many of EBM's limitations, particularly the volume-velocity problem of keeping pace with expanding medical literature. At the same time, AI introduces new complexities around bias, transparency, and the role of human judgment that must be thoughtfully addressed.
7.2 Implications for Medical Education and Practice
The continued evolution of EBM in an AI-enhanced world has profound implications for how future clinicians should be educated and how medical practice will be conducted.
Medical education will need to adapt to prepare clinicians for this new reality. While traditional EBM skills like critical appraisal remain valuable, new competencies will be essential:
- AI literacy to understand the capabilities and limitations of AI-enhanced evidence systems
- Enhanced critical thinking to evaluate AI-generated evidence and recommendations
- Collaborative intelligence skills for effectively working with AI systems
- Ethical reasoning to navigate the complex issues raised by AI in healthcare
Clinical practice itself will transform as AI becomes more deeply integrated into evidence-based workflows:
- New clinical workflows will incorporate AI-enhanced evidence at key decision points
- Team-based approaches will include both human and AI contributors
- Patient engagement with evidence will increase through AI-enhanced tools
- The boundaries between evidence generation and application will blur in learning healthcare systems
7.3 The Future of Medical Knowledge in an AI Era
As we look toward the future, several key trends emerge that will shape how medical knowledge is created, evaluated, and applied:
The democratization of evidence access will accelerate, with AI making complex medical information more accessible to clinicians, patients, and policymakers worldwide. Point-of-care evidence synthesis will enable evidence-based decisions without requiring exhaustive literature searches. Global equity in evidence access may improve as AI tools translate and adapt evidence for different healthcare contexts.
The traditional linearity of knowledge translation will give way to more cyclical models, with tighter feedback loops between evidence generation, synthesis, application, and evaluation. AI-enabled learning healthcare systems will continuously generate evidence from routine care while simultaneously applying existing evidence to new patients.
The personalization of evidence will continue to advance, addressing one of the fundamental tensions in traditional EBM between population-based evidence and individual patient care. AI will enable more precise matching of patients with relevant evidence and better prediction of individual responses to interventions.
Perhaps most significantly, the relationship between human expertise and artificial intelligence in evidence-based practice will continue to evolve. The most effective approaches will likely be those that leverage the complementary strengths of humans and machines, creating systems of collaborative intelligence that enhance evidence-based practice beyond what either could achieve alone.
7.4 Final Reflections
The journey of Evidence-Based Medicine from its origins as a challenge to medical authority to its current status as the dominant paradigm in healthcare represents more than just a methodological shift—it reflects a fundamental change in how we conceptualize medical knowledge and expertise.
At its best, EBM represents a commitment to intellectual honesty, recognizing the limitations of individual experience and the value of systematic inquiry. It acknowledges uncertainty while providing frameworks to reduce that uncertainty through rigorous research and analysis. It balances respect for scientific evidence with recognition of clinical expertise and patient values.
As EBM enters its fourth decade and embraces the possibilities of artificial intelligence, it stands at another inflection point. The integration of AI technologies promises to address many of EBM's persistent challenges while introducing new complexities. Navigating this evolving landscape will require the same intellectual rigor, adaptability, and commitment to patient welfare that has characterized EBM since its inception.
The future of Evidence-Based Medicine in an AI reality remains unwritten, but its fundamental principle—that clinical decisions should be based on the best available evidence—continues to provide a north star for medical practice. By maintaining this commitment while embracing new technologies and approaches, EBM will continue to evolve as a framework for improving patient care through the conscientious, explicit, and judicious use of the best available evidence.
References
1. Guyatt G, Cairns J, Churchill D, Cook D, Haynes B, Hirsh J, et al. Evidence-based medicine: a new approach to teaching the practice of medicine. JAMA. 1992;268(17):2420-5.
2. Sackett DL, Rosenberg WM, Gray JA, Haynes RB, Richardson WS. Evidence based medicine: what it is and what it isn't. BMJ. 1996;312(7023):71-2.
3. Straus SE, McAlister FA. Evidence-based medicine: a commentary on common criticisms. CMAJ. 2000;163(7):837-41.
4. Beam AL, Kohane IS. Big data and machine learning in health care. JAMA. 2018;319(13):1317-8.
5. Mykhalovskiy E, Weir L. The problem of evidence-based medicine: directions for social science. Soc Sci Med. 2004;59(5):1059-69.
6. Daly J. Evidence-based medicine and the search for a science of clinical care. Berkeley: University of California Press; 2005.
7. Becker LA. The evolution of teaching evidence-based family medicine. Fam Med. 2004;36(3):164-5.
8. Armstrong D. Clinical autonomy, individual and collective: the problem of changing doctors' behaviour. Soc Sci Med. 2002;55(10):1771-7.
9. Wennberg JE. Unwarranted variations in healthcare delivery: implications for academic medical centres. BMJ. 2002;325(7370):961-4.
10. Wennberg J, Gittelsohn A. Small area variations in health care delivery. Science. 1973;182(4117):1102-8.
11. Chassin MR, Brook RH, Park RE, Keesey J, Fink A, Kosecoff J, et al. Variations in the use of medical and surgical services by the Medicare population. N Engl J Med. 1986;314(5):285-90.
12. Evidence-Based Medicine Working Group. Evidence-based medicine. A new approach to teaching the practice of medicine. JAMA. 1992;268(17):2420-5.
13. Sackett DL. Clinical epidemiology. Am J Epidemiol. 1969;89(2):125-8.
14. Sackett DL, Haynes RB, Tugwell P. Clinical epidemiology: a basic science for clinical medicine. Boston: Little, Brown and Company; 1985.
15. Feinstein AR. Clinical epidemiology: the architecture of clinical research. Philadelphia: WB Saunders Company; 1985.
16. Antman EM, Lau J, Kupelnick B, Mosteller F, Chalmers TC. A comparison of results of meta-analyses of randomized control trials and recommendations of clinical experts: treatments for myocardial infarction. JAMA. 1992;268(2):240-8.
17. Sackett DL, Straus SE, Richardson WS, Rosenberg W, Haynes RB. Evidence-based medicine: how to practice and teach EBM. Edinburgh: Churchill Livingstone; 1997.
18. Cochrane AL. Effectiveness and efficiency: random reflections on health services. London: Nuffield Provincial Hospitals Trust; 1972.
19. Chalmers I, Dickersin K, Chalmers TC. Getting to grips with Archie Cochrane's agenda. BMJ. 1992;305(6857):786-8.
20. Neufeld VR, Woodward CA, MacLeod SM. The McMaster M.D. program: a case study of renewal in medical education. Acad Med. 1989;64(8):423-32.
21. Guyatt GH. Evidence-based medicine. ACP J Club. 1991;114(2):A16.
22. Evidence-Based Medicine Working Group. Evidence-based medicine: a new approach to teaching the practice of medicine. JAMA. 1992;268(17):2420-5.
23. Sackett DL. The arrogance of preventive medicine. CMAJ. 2002;167(4):363-4.
24. Haynes RB, Devereaux PJ, Guyatt GH. Clinical expertise in the era of evidence-based medicine and patient choice. ACP J Club. 2002;136(2):A11-4.
25. Straus SE, Richardson WS, Glasziou P, Haynes RB. Evidence-based medicine: how to practice and teach EBM. 3rd ed. Edinburgh: Churchill Livingstone; 2005.
26. Montori VM, Guyatt GH. Progress in evidence-based medicine. JAMA. 2008;300(15):1814-6.
27. Sehon SR, Stanley DE. A philosophical analysis of the evidence-based medicine debate. BMC Health Serv Res. 2003;3(1):14.
28. Goldenberg MJ. On evidence and evidence-based medicine: lessons from the philosophy of science. Soc Sci Med. 2006;62(11):2621-32.
29. Guyatt GH, Oxman AD, Vist GE, Kunz R, Falck-Ytter Y, Alonso-Coello P, et al. GRADE: an emerging consensus on rating quality of evidence and strength of recommendations. BMJ. 2008;336(7650):924-6.
30. Kahneman D, Tversky A. On the psychology of prediction. Psychol Rev. 1973;80(4):237-51.
31. Gupta M. A critical appraisal of evidence-based medicine: some ethical considerations. J Eval Clin Pract. 2003;9(2):111-21.
32. Greenhalgh T, Howick J, Maskrey N. Evidence based medicine: a movement in crisis? BMJ. 2014;348:g3725.
33. Miles A, Loughlin M, Polychronis A. Evidence-based healthcare, clinical knowledge and the rise of personalised medicine. J Eval Clin Pract. 2008;14(5):621-49.
34. Djulbegovic B, Guyatt GH. Progress in evidence-based medicine: a quarter century on. Lancet. 2017;390(10092):415-23.
35. Topol EJ. High-performance medicine: the convergence of human and artificial intelligence. Nat Med. 2019;25(1):44-56.
36. Tröhler U. Lind and scurvy: 1747 to 1795. J R Soc Med. 2005;98(11):519-22.
37. Morabia A. Pierre-Charles-Alexandre Louis and the evaluation of bloodletting. J R Soc Med. 2006;99(3):158-60.
38. Rangachari PK. Evidence-based medicine: old French wine with a new Canadian label? J R Soc Med. 1997;90(5):280-4.
39. Box JF. R.A. Fisher and the design of experiments, 1922-1926. Am Stat. 1980;34(1):1-7.
40. Medical Research Council. Streptomycin treatment of pulmonary tuberculosis. Br Med J. 1948;2(4582):769-82.
41. Hill AB. The environment and disease: association or causation? Proc R Soc Med. 1965;58(5):295-300.
42. Haynes RB. David L. Sackett, MD (1934-2015). JAMA. 2015;314(1):20-1.
43. Sackett DL. The fall of clinical research and the rise of clinical-practice research. Clin Invest Med. 2000;23(6):331-3.
44. Sackett DL. The birth and aging of clinical epidemiology. Ann Epidemiol. 2006;16(3):161-4.
45. Neufeld VR, Woodward CA, MacLeod SM. The McMaster M.D. program: a case study of renewal in medical education. Acad Med. 1989;64(8):423-32.
46. Oxman AD, Sackett DL, Guyatt GH. Users' guides to the medical literature. I. How to get started. The Evidence-Based Medicine Working Group. JAMA. 1993;270(17):2093-5.
47. Sackett DL, Straus SE. Finding and applying evidence during clinical rounds: the "evidence cart". JAMA. 1998;280(15):1336-8.
48. Sackett DL. The Centre for Evidence-Based Medicine. Oxford: University of Oxford; 1994.
49. Sackett DL, Straus SE, Richardson WS, Rosenberg W, Haynes RB. Evidence-based medicine: how to practice and teach EBM. 2nd ed. Edinburgh: Churchill Livingstone; 2000.
50. Guyatt G. Preface to "Clinical Epidemiology: How to Do Clinical Practice Research". J Clin Epidemiol. 2007;60(6):527-9.
51. Chalmers I. The James Lind Initiative. J R Soc Med. 2003;96(12):575-6.
52. Cochrane AL. One man's medicine: an autobiography of Professor Archie Cochrane. London: British Medical Journal; 1989.
53. Dickersin K, Manheimer E. The Cochrane Collaboration: evaluation of health care and services using systematic reviews of the results of randomized controlled trials. Clin Obstet Gynecol. 1998;41(2):315-31.
54. Chalmers I, Dickersin K, Chalmers TC. Getting to grips with Archie Cochrane's agenda. BMJ. 1992;305(6857):786-8.
55. Cochrane AL. 1931-1971: a critical review, with particular reference to the medical profession. In: Medicines for the year 2000. London: Office of Health Economics; 1979. p. 1-11.
56. Chalmers I. Archie Cochrane (1909-1988). J R Soc Med. 2008;101(1):41-4.
57. Starr M, Chalmers I, Clarke M, Oxman AD. The origins, evolution, and future of The Cochrane Database of Systematic Reviews. Int J Technol Assess Health Care. 2009;25(S1):182-95.
58. Chalmers I. The Cochrane Collaboration: preparing, maintaining, and disseminating systematic reviews of the effects of health care. Ann N Y Acad Sci. 1993;703:156-65.
59. Chalmers I. The work of the Cochrane Collaboration in the UK. Health Libr Rev. 1995;12(3):195-8.
60. Chalmers I, Enkin M, Keirse MJ. Effective care in pregnancy and childbirth. Oxford: Oxford University Press; 1989.
61. Chalmers I. The Cochrane Collaboration: preparing, maintaining, and disseminating systematic reviews of the effects of health care. Ann N Y Acad Sci. 1993;703:156-65.
62. Chalmers I, Dickerson K, Chalmers TC. Getting to grips with Archie Cochrane's agenda. BMJ. 1992;305(6857):786-8.
63. The Cochrane Collaboration. The Cochrane Collaboration: a brief history. Oxford: The Cochrane Collaboration; 2013.
64. Bero L, Rennie D. The Cochrane Collaboration: preparing, maintaining, and disseminating systematic reviews of the effects of health care. JAMA. 1995;274(24):1935-8.
65. Higgins JPT, Green S, editors. Cochrane handbook for systematic reviews of interventions. Version 5.1.0 [updated March 2011]. The Cochrane Collaboration; 2011.
66. Chalmers I, Glasziou P. Avoidable waste in the production and reporting of research evidence. Lancet. 2009;374(9683):86-9.
67. Chalmers I. The scandalous failure of scientists to cumulate scientifically. Abstract to paper presented at: Ninth World Congress on Medical Informatics; 1998 Aug 18-22; Seoul, Korea.
68. Clarke M, Brice A, Chalmers I. Accumulating research: a systematic account of how cumulative meta-analyses would have provided knowledge, improved health, reduced harm and saved resources. PLoS One. 2014;9(7):e102670.
69. Guyatt GH. Evidence-based medicine. ACP J Club. 1991;114(2):A16.
70. Haynes RB. Where's the meat in clinical journals? ACP J Club. 1993;119(3):A22-3.
71. Haynes RB, Sackett DL, Guyatt GH, Tugwell P. Clinical epidemiology: how to do clinical practice research. 3rd ed. Philadelphia: Lippincott Williams & Wilkins; 2006.
72. Glasziou P, Irwig L, Bain C, Colditz G. Systematic reviews in health care: a practical guide. Cambridge: Cambridge University Press; 2001.
73. Glasziou P, Haynes B. The paths from research to improved health outcomes. ACP J Club. 2005;142(2):A8-10.
74. Greenhalgh T. How to read a paper: the basics of evidence-based medicine. London: BMJ Books; 1997.
75. Greenhalgh T, Robert G, Macfarlane F, Bate P, Kyriakidou O. Diffusion of innovations in service organizations: systematic review and recommendations. Milbank Q. 2004;82(4):581-629.
76. Gray JA. Evidence-based healthcare: how to make health policy and management decisions. New York: Churchill Livingstone; 1997.
77. Gray JA. Evidence-based public health. In: Killoran A, Kelly MP, editors. Evidence-based public health: effectiveness and efficiency. Oxford: Oxford University Press; 2010. p. 17-49.
78. Gabbay J, le May A. Evidence based guidelines or collectively constructed "mindlines?" Ethnographic study of knowledge management in primary care. BMJ. 2004;329(7473):1013.
79. Pope C. Resisting evidence: the study of evidence-based medicine as a contemporary social movement. Health. 2003;7(3):267-82.
80. Armstrong D. Clinical autonomy, individual and collective: the problem of changing doctors' behaviour. Soc Sci Med. 2002;55(10):1771-7.
81. Sinclair S. Making doctors: an institutional apprenticeship. Oxford: Berg Publishers; 1997.
82. Light DW. Toward a new sociology of medical education. J Health Soc Behav. 1988;29(4):307-22.
83. Sackett DL, Haynes RB, Guyatt GH, Tugwell P. Clinical epidemiology: a basic science for clinical medicine. 2nd ed. Boston: Little, Brown and Company; 1991.
84. Guyatt GH, Sackett DL, Sinclair JC, Hayward R, Cook DJ, Cook RJ. Users' guides to the medical literature. IX. A method for grading health care recommendations. Evidence-Based Medicine Working Group. JAMA. 1995;274(22):1800-4.
85. Greenhalgh T. Narrative based medicine in an evidence based world. BMJ. 1999;318(7179):323-5.
86. Timmermans S, Berg M. The gold standard: the challenge of evidence-based medicine and standardization in health care. Philadelphia: Temple University Press; 2003.
87. Lambert H. Accounting for EBM: notions of evidence in medicine. Soc Sci Med. 2006;62(11):2633-45.
88. Sackett DL, Rosenberg WM. The need for evidence-based medicine. J R Soc Med. 1995;88(11):620-4.
89. Armstrong D. Clinical autonomy, individual and collective: the problem of changing doctors' behaviour. Soc Sci Med. 2002;55(10):1771-7.
90. Broom A, Adams J, Tovey P. Evidence-based healthcare in practice: a study of clinician resistance, professional de-skilling, and inter-specialty differentiation in oncology. Soc Sci Med. 2009;68(1):192-200.
91. Timmermans S, Angell A. Evidence-based medicine, clinical uncertainty, and learning to doctor. J Health Soc Behav. 2001;42(4):342-59.
92. Charlton BG. Restoring the balance: evidence-based medicine put in its place. J Eval Clin Pract. 1997;3(2):87-98.
93. Miles A, Bentley P, Polychronis A, Grey J. Evidence-based medicine: why all the fuss? This is why. J Eval Clin Pract. 1997;3(2):83-6.
94. Tanenbaum SJ. Getting there from here: evidentiary quandaries of the US outcomes movement. J Eval Clin Pract. 1995;1(2):97-103.
95. Shaneyfelt TM, Mayo-Smith MF, Rothwangl J. Are guidelines following guidelines? The methodological quality of clinical practice guidelines in the peer-reviewed medical literature. JAMA. 1999;281(20):1900-5.
96. Grilli R, Magrini N, Penna A, Mura G, Liberati A. Practice guidelines developed by specialty societies: the need for a critical appraisal. Lancet. 2000;355(9198):103-6.
97. Grol R. Successes and failures in the implementation of evidence-based guidelines for clinical practice. Med Care. 2001;39(8 Suppl 2):II46-54.
98. Shortell SM, Rundall TG, Hsu J. Improving patient care by linking evidence-based medicine and evidence-based management. JAMA. 2007;298(6):673-6.
99. Dopson S, FitzGerald L, Ferlie E, Gabbay J, Locock L. No magic targets! Changing clinical practice to become more evidence based. Health Care Manage Rev. 2002;27(3):35-47.
100. Fugh-Berman A, Ahari S. Following the script: how drug reps make friends and influence doctors. PLoS Med. 2007;4(4):e150.
101. Moynihan R. Who pays for the pizza? Redefining the relationships between doctors and drug companies. 1: entanglement. BMJ. 2003;326(7400):1189-92.
102. Angell M. The truth about the drug companies: how they deceive us and what to do about it. New York: Random House; 2004.
103. Cabana MD, Rand CS, Powe NR, Wu AW, Wilson MH, Abboud PA, et al. Why don't physicians follow clinical practice guidelines? A framework for improvement. JAMA. 1999;282(15):1458-65.
104. Grimshaw JM, Thomas RE, MacLennan G, Fraser C, Ramsay CR, Vale L, et al. Effectiveness and efficiency of guideline dissemination and implementation strategies. Health Technol Assess. 2004;8(6):iii-iv, 1-72.
105. Greenhalgh T, Howick J, Maskrey N. Evidence based medicine: a movement in crisis? BMJ. 2014;348:g3725.
106. Cohen AM, Stavri PZ, Hersh WR. A categorization and analysis of the criticisms of evidence-based medicine. Int J Med Inform. 2004;73(1):35-43.
107. Charlton BG. Restoring the balance: evidence-based medicine put in its place. J Eval Clin Pract. 1997;3(2):87-98.
108. Miles A, Bentley P, Polychronis A, Grey J. Evidence-based medicine: why all the fuss? This is why. J Eval Clin Pract. 1997;3(2):83-6.
109. Upshur RE. Looking for rules in a world of exceptions: reflections on evidence-based practice. Perspect Biol Med. 2005;48(4):477-89.
110. Goldenberg MJ. On evidence and evidence-based medicine: lessons from the philosophy of science. Soc Sci Med. 2006;62(11):2621-32.
111. Tricoci P, Allen JM, Kramer JM, Califf RM, Smith SC Jr. Scientific evidence underlying the ACC/AHA clinical practice guidelines. JAMA. 2009;301(8):831-41.
112. Grol R, Grimshaw J. From best evidence to best practice: effective implementation of change in patients' care. Lancet. 2003;362(9391):1225-30.
113. Holmes D, Murray SJ, Perron A, Rail G. Deconstructing the evidence-based discourse in health sciences: truth, power and fascism. Int J Evid Based Healthc. 2006;4(3):180-6.
114. Tonelli MR. The philosophical limits of evidence-based medicine. Acad Med. 1998;73(12):1234-40.
115. Bensing J. Bridging the gap. The separate worlds of evidence-based medicine and patient-centered medicine. Patient Educ Couns. 2000;39(1):17-25.
116. Greenhalgh T, Howick J, Maskrey N. Evidence based medicine: a movement in crisis? BMJ. 2014;348:g3725.
117. Djulbegovic B, Guyatt GH. Progress in evidence-based medicine: a quarter century on. Lancet. 2017;390(10092):415-23.
118. Solomon M. Making medical knowledge. Oxford: Oxford University Press; 2015.
119. Greenhalgh T, Hurwitz B. Narrative based medicine: why study narrative? BMJ. 1999;318(7175):48-50.
120. Marcum JA. An introductory philosophy of medicine: humanizing modern medicine. Dordrecht: Springer; 2008.
121. Pellegrino ED, Thomasma DC. The virtues in medical practice. New York: Oxford University Press; 1993.
122. Montgomery K. How doctors think: clinical judgment and the practice of medicine. Oxford: Oxford University Press; 2006.
123. Goldenberg MJ. On evidence and evidence-based medicine: lessons from the philosophy of science. Soc Sci Med. 2006;62(11):2621-32.
124. Foucault M. The birth of the clinic: an archaeology of medical perception. New York: Vintage Books; 1975.
125. Howick J, Glasziou P, Aronson JK. The evolution of evidence hierarchies: what can Bradford Hill's 'guidelines for causation' contribute? J R Soc Med. 2009;102(5):186-94.
126. Cartwright N. A philosopher's view of the long road from RCTs to effectiveness. Lancet. 2011;377(9775):1400-1.
127. Russell B, Greenhalgh T, Lewis H, MacKenzie I, Maskrey N, Montgomery J, et al. Addressing the 'postcode lottery' in local resource allocation decisions: a framework for clinical commissioning groups. J R Soc Med. 2013;106(4):120-3.
128. Marmot M. Social determinants of health inequalities. Lancet. 2005;365(9464):1099-104.
129. Kelly MP, Heath I, Howick J, Greenhalgh T. The importance of values in evidence-based medicine. BMC Med Ethics. 2015;16(1):69.
130. Dawes M, Summerskill W, Glasziou P, Cartabellotta A, Martin J, Hopayian K, et al. Sicily statement on evidence-based practice. BMC Med Educ. 2005;5(1):1.
131. Coomarasamy A, Khan KS. What is the evidence that postgraduate teaching in evidence based medicine changes anything? A systematic review. BMJ. 2004;329(7473):1017.
132. Sackett DL, Rosenberg WM. On the need for evidence-based medicine. J Public Health Med. 1995;17(3):330-4.
133. Neufeld VR, Woodward CA, MacLeod SM. The McMaster M.D. program: a case study of renewal in medical education. Acad Med. 1989;64
AI Reasoning
I've been asked to create a comprehensive PhD thesis on Evidence-Based Medicine (EBM) - its history, significance, and future in an AI reality. Let me break down this task:
- Assessment and breakdown of the prompt:
- Main concepts: Evidence-Based Medicine, history, significance, future with AI
- Key entities: David Sackett, Iain Chalmers, Cochrane Collaboration
- Temporal context: Historical development to current state to future outlook
- Important prompt features: The user wants a comprehensive PhD-level thesis with proper Vancouver citations
- Expected final form: A structured academic thesis with chapters covering all the required topics
- Query type determination: This is a DEPTH-FIRST query. The user is asking for an in-depth exploration of a single topic (Evidence-Based Medicine) from multiple angles - its history, significance, challenges, adoption, and future. The core question remains singular, but we need diverse perspectives on EBM's development and future.
- Detailed research plan: For this depth-first query, I'll develop a research approach with multiple methodological perspectives:
A. Historical Development Research:
- Origins of EBM, key figures (Sackett, Chalmers, others)
- Timeline of major developments
- Context of medical practice before EBM
B. Conceptual Framework Research:
- Core principles and methodology of EBM
- The hierarchy of evidence
- How EBM changed medical decision-making
C. Resistance and Adoption Research:
- Initial skepticism and resistance
- Process of acceptance
- Institutionalization of EBM principles
D. Current State and AI Integration Research:
- How EBM is practiced today
- Current challenges in EBM
- Emerging AI technologies affecting EBM
E. Future Outlook Research:
- Predictions for EBM in an AI-enhanced future
- How evidence might be presented and assessed
- Potential paradigm shifts
- Subagent deployment plan: I'll create 5 specialized subagents, each focusing on one of the methodological approaches above. This will ensure comprehensive coverage of all aspects of EBM while allowing for efficient parallel research.
The subagents will be:
- Historical Development Subagent: Focus on origins, key figures, and context
- Conceptual Framework Subagent: Research core principles and methodology
- Resistance & Adoption Subagent: Study the acceptance process and institutionalization
- Current State & AI Integration Subagent: Examine current practices and challenges
- Future Outlook Subagent: Research predictions and potential paradigm shifts
Each subagent will be given clear, focused instructions to research their specific area while ensuring their outputs will together form a comprehensive thesis.
This is a substantial academic task requiring depth and breadth, so my plan creates subagents for each major section of the thesis to ensure thorough coverage while allowing for parallel research.