Table of Contents
Chapter 1: Introduction
Evidence-Based Medicine (EBM) emerged in the late 20th century as a transformative approach to clinical practice, emphasizing the use of the best available evidence from systematic research in making medical decisions. It represented a shift from the traditional reliance on authority and anecdote towards a more scientific, data-driven paradigm. In the three decades since its introduction, EBM has fundamentally changed how physicians learn, practice, and evaluate medicine. It has given rise to new institutions (such as the Cochrane Collaboration), new methodologies (systematic reviews and meta-analyses), and a culture of continual questioning and appraisal of the evidence behind clinical interventions. This doctoral thesis provides a comprehensive exploration of EBM’s evolution: from its historical foundations and key figures, through its principles and impact on clinical care and health policy, and finally to its future in the era of artificial intelligence (AI).
The thesis begins with a historical overview of EBM, highlighting the roles of pioneers like David Sackett and Iain Chalmers in shaping the movement. It then outlines the core principles and methodologies of EBM, examining how this approach has been applied across medical disciplines to improve clinical decision-making and patient outcomes. The transformative impact of EBM on day-to-day patient care and on broader health policy is analyzed, illustrating how it has improved quality of care and informed guidelines and decision-making processes at institutional and policy levels.
In the latter part, a full chapter is dedicated to current and emerging applications of AI in evidence-based practice. AI technologies – from machine learning predictive models to natural language processing tools for literature review – promise to further revolutionize EBM by handling complex data and automating parts of the evidence synthesis and decision process. We explore how AI is being integrated into EBM, for example in predictive modeling for patient risk stratification, automation of systematic reviews, and intelligent clinical decision support systems. A critical discussion follows on the epistemological and ethical implications of incorporating AI into EBM: How might AI-generated insights be evaluated as evidence? What are the risks of bias, and how can transparency and trust be maintained in an AI-augmented evidence ecosystem?
By examining EBM’s past, present, and future, this thesis aims to demonstrate that evidence-based practice is not a static set of rules but a dynamic and evolving paradigm. As medicine enters an age of big data and AI, the foundational ethos of EBM – making decisions based on the conscientious, explicit, and judicious use of current best evidence – remains as crucial as ever. The integration of AI into EBM, if guided by rigorous validation and ethical oversight, has the potential to inaugurate a new era of “evidence-based healthcare 2.0”, improving the efficiency, personalization, and impact of medical care. The concluding chapter offers perspectives on how clinicians, researchers, and policymakers can harness AI while upholding the principles of EBM to ensure that the future of medicine remains firmly evidence-based and patient-centered.
Chapter 2: Historical Foundations of Evidence-Based Medicine
2.1 Origins of a Movement – The Late 20th Century Medical Context
In the mid-20th century, clinical medicine largely depended on expert opinion, clinical experience, and pathophysiological rationale as the basis for practice. While scientific research and clinical trials existed, their results were not systematically integrated into everyday care. Pioneering voices began to question this state of affairs. One notable figure was Archibald L. Cochrane, a British epidemiologist whose seminal 1972 book Effectiveness and Efficiency: Random Reflections on Health Services argued for the critical assessment of medical interventions. Cochrane highlighted the lack of reliable summaries of evidence, famously stating in 1979 that “it is surely a great criticism of our profession that we have not organised a critical summary, by specialty or subspecialty, adapted periodically, of all relevant randomized controlled trials”. This pointed critique underscored the need for organized, up-to-date syntheses of research, planting a seed that would later grow into the systematic review enterprise of EBM.
Around the same period, health services researchers like John Wennberg in the United States uncovered striking variations in medical practice patterns that could not be explained by patient populations or preferences. Wennberg’s studies in the 1970s showed, for example, that certain surgeries (such as tonsillectomies or prostatectomies) were performed at markedly different rates in demographically similar populations. The logical explanation was that these differences stemmed from local practice habits or physicians’ beliefs rather than from solid evidence – in other words, a lack of a common evidence-based standard of care. Such findings further propelled the idea that medicine needed a more rigorous, empirical foundation. In 1990, physician David M. Eddy introduced the term “evidence-based” in a health policy context, using it to emphasize how many common practices lacked scientific backing. By highlighting that “if the majority of physicians were doing it, it was considered medically necessary” regardless of evidence, Eddy called attention to a tautology that EBM would seek to break.
2.2 David Sackett and the Birth of Clinical Epidemiology
While the intellectual climate was shifting, a more formal development of what would become EBM was taking shape in Canada. Dr. David L. Sackett (1934–2015), often regarded as the “father of evidence-based medicine”, was instrumental in this evolution. In 1967, Sackett founded the world’s first Department of Clinical Epidemiology and Biostatistics at McMaster University in Hamilton, Ontario. This new department was dedicated to applying epidemiological methods to clinical research and training a generation of physicians to critically appraise medical literature. Sackett and colleagues developed ways to teach medical trainees how to frame clinical questions, search for relevant research, and interpret study results – essentially creating the toolkit for practicing medicine based on evidence rather than solely on intuition or tradition.
By the late 1980s, these ideas coalesced into a clear concept. At McMaster University, a group of physicians led by Sackett and Dr. Gordon Guyatt began using the term “Evidence-Based Medicine” to describe this new approach to clinical practice and teaching. Guyatt coined the term “evidence-based medicine” around 1990 in an internal document for McMaster’s residency program, and it first appeared in print in 1991 in an editorial for the ACP Journal Club. The concept gained international attention with the publication of the landmark paper “Evidence-Based Medicine: A New Approach to Teaching the Practice of Medicine” by the Evidence-Based Medicine Working Group in the Journal of the American Medical Association (JAMA) in 1992. This publication outlined a paradigm in which clinical decisions should be based on the integration of best research evidence with clinical expertise and patient values, and it provided a framework for how clinicians could learn and apply these skills.
David Sackett continued to champion EBM through both practice and scholarship. In 1994, he moved to Oxford University to establish the Centre for Evidence-Based Medicine, further spreading the movement internationally. Sackett authored (with colleagues) the seminal 1996 BMJ article “Evidence based medicine: what it is and what it isn’t,” which offered a concise definition of EBM that remains widely cited. In it, EBM is defined as “the conscientious, explicit, and judicious use of current best evidence in making decisions about the care of individual patients”, and that “the practice of EBM means integrating individual clinical expertise with the best available external clinical evidence from systematic research”. This definition underscored that EBM was not “cookbook medicine” ignoring clinician judgment; rather, it was a way to enhance clinical decision-making by grounding it in reliable evidence.
Under Sackett’s influence and that of like-minded educators, the 1990s saw an explosion of EBM resources and training. Academic centers around the world began incorporating EBM principles into curricula. By 1997, just five years after the term entered the mainstream, over a thousand articles per year were being published that referenced “evidence-based medicine”, dedicated EBM journals and textbooks had appeared, and clinicians were forming journal clubs to practice critical appraisal. Sackett himself authored textbooks such as Evidence-Based Medicine: How to Practice and Teach EBM, and helped launch journals (e.g., Evidence-Based Medicine and ACP Journal Club) devoted to summarizing evidence for practitioners. This rapid uptake marked a profound shift in medical culture, from one in which deference to senior physicians’ experience was paramount to one in which even a junior clinician could question practice by asking, “What is the evidence for this?”.
2.3 Iain Chalmers and the Cochrane Collaboration
While Sackett was advancing EBM in clinical training and practice, another key figure, Sir Iain Chalmers, was revolutionizing the way evidence was summarized and synthesized. A British health services researcher, Chalmers was driven by the belief that robust evidence should drive healthcare decisions, and that means gathering all relevant data to understand what works and what doesn’t. In the late 1970s and 1980s, Chalmers focused on improving maternity care by systematically reviewing evidence from clinical trials in pregnancy and childbirth. As director of the National Perinatal Epidemiology Unit in Oxford (from 1978 to 1992), he led the development of the Oxford Database of Perinatal Trials, one of the first electronic repositories of trial data in medicine. He also co-edited Effective Care in Pregnancy and Childbirth (1989), a two-volume collection of systematic reviews that set new standards for how to compile and assess evidence in a medical specialty.
Chalmers was directly inspired by Archie Cochrane’s call for organized, up-to-date summaries of evidence. Building on this inspiration and his own experience in perinatal care, Chalmers sought to expand systematic reviewing to all areas of health care. In 1992, he was appointed as the first director of the UK Cochrane Centre. The following year, in October 1993, an international gathering of 77 scholars and clinicians from multiple countries met in Oxford for the inaugural Cochrane Colloquium. There, the Cochrane Collaboration was formally launched – a global, independent network with the mission of preparing, maintaining, and disseminating systematic reviews of healthcare interventions, in honor of Archie Cochrane’s legacy.
The founding of the Cochrane Collaboration in 1993 under Chalmers’ leadership marked a watershed moment in the history of EBM. For the first time, there was an organized international effort to regularly collect and summarize evidence from clinical trials on a worldwide scale. Initially focusing on areas like pregnancy and childbirth (where Chalmers had demonstrated the approach), Cochrane soon expanded to cover all specialties. By establishing rigorous methods for literature search, data extraction, and meta-analysis, and by updating reviews as new data emerged (the concept of “living” reviews), the Cochrane Collaboration greatly advanced the reliability of the evidence base available to clinicians and policymakers. Chalmers and colleagues adopted principles of openness, collaboration, and avoidance of duplication – inviting researchers globally to contribute to a shared effort rather than work in silos. The result was a steadily growing repository of high-quality systematic reviews (published initially in the Cochrane Database of Systematic Reviews), which soon became a cornerstone of EBM.
By the 2000s, the impact of Cochrane was evident. National and international guideline bodies increasingly looked to Cochrane reviews as gold-standard syntheses of evidence. Clinicians had access (often free or through institutions) to summaries of evidence that would have been impractical to assemble on their own. The collaboration’s ethos also spread: many other organizations and journals began to produce systematic reviews, adopting methods either developed or popularized by Cochrane. In essence, Cochrane operationalized one of the central visions of EBM – making the “current best evidence” readily available and continually updated for use in practice.
It is worth noting that EBM’s historical development was a collaborative and international effort. Aside from Sackett and Chalmers, numerous other contributors helped shape the movement. Gordon Guyatt, as mentioned, played a key role in articulating and disseminating EBM concepts. Brian Haynes, Archie Cochrane, Alan Oxman, Paul Glasziou, Trish Greenhalgh, and many others made contributions ranging from developing critical appraisal checklists to integrating evidence-based guidelines into clinical workflows. By the turn of the 21st century, evidence-based medicine had transformed from a novel idea into a broad movement. The phrase “EBM” itself had become part of the medical lexicon, symbolizing a new norm: that clinical practice should be continually informed by high-quality evidence and that both training and research should focus on finding and applying what actually works for patients.
Chapter 3: Principles and Methodology of EBM
3.1 Core Principles: Integrating Evidence, Expertise, and Patient Values
At its heart, Evidence-Based Medicine is about using evidence to inform medical decisions. But crucially, as defined by Sackett et al., it is the integration of three components: best available external evidence, clinical expertise, and patient values/preferences. This triad ensures that EBM is not a one-size-fits-all algorithm, but a balanced approach:
- Best External Evidence refers to clinically relevant research, often from patient-centered studies such as randomized controlled trials (RCTs) or observational cohort studies, ideally synthesized in systematic reviews and meta-analyses. EBM gives primacy to evidence that is systematically collected and free from biases to the extent possible. Not all evidence is equal – hence the development of evidence hierarchies (discussed below). The goal is to find the highest-quality evidence available to answer a specific clinical question.
- Clinical Expertise refers to the proficiency and judgment that clinicians acquire through experience and practice. A skilled clinician can assess the patient’s situation, interpret the evidence in context, and determine whether and how it applies. EBM explicitly acknowledges that evidence alone is never enough; it must be filtered through the lens of the clinician’s own expertise in diagnosis, risk assessment, and communication. Experienced clinicians provide insight into the feasibility of interventions and can recognize when published evidence might not apply due to a patient’s unique circumstances or comorbidities.
- Patient Values and Preferences are the third crucial pillar. Good medical care involves understanding the patient’s individual circumstances, goals, and preferences. Two patients with the same medical condition might make different choices when presented with the risks and benefits of treatment options, depending on what outcomes matter most to them. EBM encourages shared decision-making, where clinicians use evidence to inform patients and then incorporate the patient’s values in the final decision. As one formulation puts it, evidence-based practice means decisions are based on “three sources of information: evidence, clinical experience, and patient preferences”.
These principles address a key misconception: EBM is not meant to replace clinician judgment or patient input with research evidence, but to complement and enhance them. The initial rhetoric of EBM in the 1990s (“shift from intuition to evidence”) was sometimes misinterpreted as devaluing clinical experience, but in truth EBM’s thought leaders always emphasized integration. The BMJ 1996 article, for instance, notes that without clinical expertise, practice risks becoming tyrannized by evidence – which can be out of context or not applicable to the individual – and without the best evidence, practice can quickly become outdated. Thus, the goal is a conscientious, explicit, and judicious use of evidence, tailored by clinical skill and patient-specific factors.
3.2 Hierarchy of Evidence and Methodological Rigor
A cornerstone of EBM methodology is the hierarchy of evidence, often visualized as a pyramid of study types ranked by their reliability in determining truth. This hierarchy stems from the principle that different study designs have different strengths and susceptibility to bias:
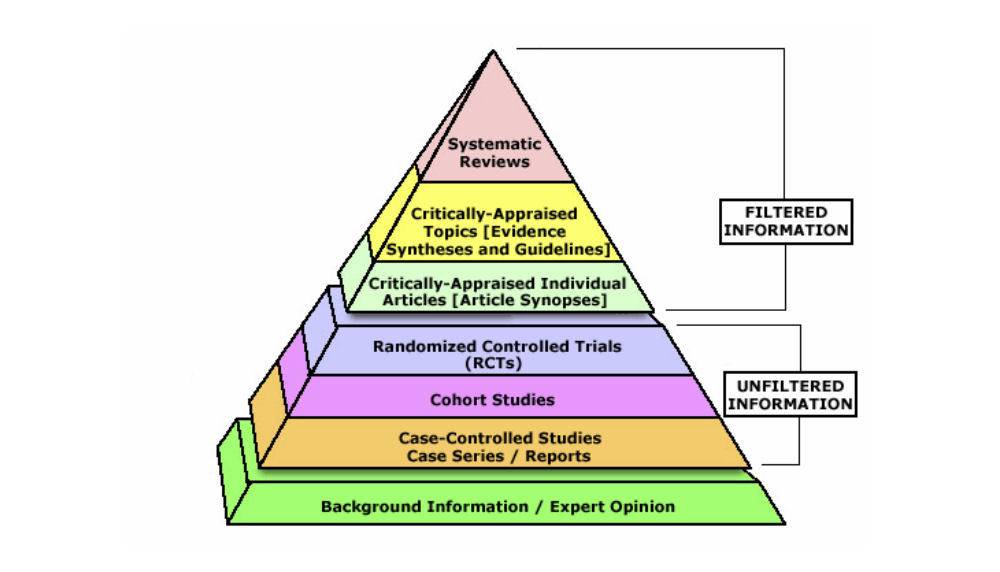
Figure 3.1: A typical Evidence-Based Medicine hierarchy of evidence pyramid. At the base are background information, expert opinion, and anecdotal experience – considered least reliable. Above that are case series and case-control studies (observational designs prone to various biases). Higher up are cohort studies (stronger observational evidence). Near the top sit randomized controlled trials (RCTs), which by randomly allocating interventions aim to minimize bias and confounding. At the apex are systematic reviews and meta-analyses of multiple studies, which synthesize all available high-quality evidence on a question, providing the strongest and most comprehensive evidence.
This hierarchy guides practitioners in weighing evidence: for questions of therapy or intervention, for example, a well-conducted RCT or a meta-analysis of RCTs provides more confidence than a single observational study or expert opinion. The pyramid is a simplification (study quality and relevance also matter), but it has been a useful educational tool in EBM. It teaches clinicians to seek the highest level of evidence available for a given question. If a systematic review exists, that might be preferable to an individual trial; if no trials exist, one might rely on observational evidence, and so on. The emphasis is on methodological rigor: evidence from a rigorously designed and executed study is valued more than evidence from a flawed one, even if they are of the same design.
EBM also brought about widespread use of critical appraisal checklists and tools. When a clinician or researcher finds a study, EBM encourages asking systematic questions about it: Was it well designed? Were patients randomly assigned? Was follow-up sufficient? Were outcomes assessed objectively? What are the confidence intervals? Tools like CASP (Critical Appraisal Skills Programme) checklists for different study types, or risk-of-bias assessment tools in systematic reviews, stem from this movement. Clinicians trained in EBM learn to read papers not just for results, but for risk of bias and applicability.
Another concept popularized by EBM is the levels of evidence in guidelines. For instance, a guideline might label a recommendation as “Level I evidence” if it’s supported by at least one high-quality randomized trial, versus “Level III” if based on expert opinion. Systems like GRADE (Grading of Recommendations Assessment, Development and Evaluation) have since formalized how to rate the quality of evidence and strength of recommendations, ensuring transparency about how confident we can be in a given recommendation based on the underlying evidence. This means that policy-makers and clinicians reading guidelines can understand whether a recommendation is on solid footing (e.g., multiple trials show benefit) or more tentative (e.g., limited evidence, or expert consensus only).
3.3 The Process of EBM in Practice – From Questions to Answers
EBM is often taught as a five-step process (sometimes summarized as the “5 A’s”):
- Ask – Formulate a clear clinical question from a patient’s problem. Typically this is done in PICO format: Patient/Problem, Intervention, Comparison, Outcome. For example: “In [elderly patients with isolated systolic hypertension] (Patient), does [treatment with a thiazide diuretic] (Intervention) compared to [no treatment] (Comparison) reduce [the risk of stroke] (Outcome)?” Formulating the question is crucial as it directs the search for evidence.
- Acquire – Search for the best available evidence. This involves knowing how to efficiently use medical databases (like PubMed, Cochrane Library) and other resources to find relevant research. The evidence hierarchy guides where to look first – for instance, one might look for a systematic review on the topic, and if none is available, then seek individual trials, etc. The advent of online databases in the 1990s, coinciding with EBM’s growth, greatly facilitated this step. Clinicians increasingly had access to Medline via the internet and could search literature during clinical rounds. EBM also fostered tools like Clinical Practice Guidelines and point-of-care references (e.g., UpToDate, which is an evidence-based summary resource) to help quickly retrieve evidence.
- Appraise – Critically appraise the evidence for its validity and relevance. Not all studies that appear in a search are equally trustworthy or applicable. In this step, clinicians evaluate whether the study methodology was sound (e.g., randomization, blinding, sample size, follow-up), what the results were (effect size, precision, statistical significance), and whether the findings are relevant to the patient in question. For example, if the patient is an 85-year-old woman, but the trial was done in 50-year-old men, one must judge how applicable the results may be. EBM training places heavy emphasis on this appraisal step, teaching concepts like Number Needed to Treat (NNT) to understand clinical significance, or likelihood ratios for diagnostic tests.
- Apply – Integrate the evidence with clinical expertise and the patient’s context and values to make a decision or recommendation. This is where the earlier steps meet real life. If evidence shows a certain therapy reduces stroke risk by 30%, the clinician must weigh that benefit against potential harms (from the evidence or experience) and consider the patient’s preferences. For instance, if the best evidence suggests a medication but the patient has a contraindication or absolutely refuses that class of drug, the clinician might opt for the second-best evidence that aligns with the patient’s situation.
- Assess – Evaluate the outcome of the decision and seek ways to improve. This feedback loop closes the EBM process. Did the patient’s outcome improve? Was the evidence applied successfully? Are there new evidence or guidelines since the decision was made? EBM advocates continuous learning – clinicians should reflect on their performance and stay alert to new research that might change practice. This step also connects to quality improvement in healthcare systems: monitoring performance metrics (like guideline adherence or patient outcomes) to ensure that evidence-based practices are truly benefiting patients.
Through these steps, EBM becomes a practical approach to clinical problem-solving. It is not restricted to academic exercises or creating guidelines; it is meant for the bedside and clinic. For example, a clinician encountering a rare condition may use the EBM approach to quickly educate themselves on management by finding a systematic review or at least case series, critically appraising it, and then discussing with the patient the best course of action given available evidence. This approach has been shown to lead to more informed and effective clinical decisions.
3.4 EBM Across Medical Disciplines and Education
One of the remarkable successes of EBM is how broadly its principles have spread across disciplines. Originally emerging in internal medicine, the EBM approach was rapidly adopted in:
- General Medicine and Primary Care: Primary care physicians were early adopters of EBM, driven by the breadth of conditions they manage and the need for efficient, evidence-based answers. The creation of evidence-based guidelines for common conditions (hypertension, diabetes, asthma, etc.) has been particularly impactful in standardizing primary care management according to evidence.
- Specialty Medicine: By the late 1990s, virtually every specialty – cardiology, oncology, surgery, psychiatry, pediatrics, you name it – had begun developing evidence-based guidelines or systematic reviews for its domain. For example, cardiology saw a revolution in care of myocardial infarction and heart failure through large RCTs, and evidence-based protocols (like giving aspirin, beta-blockers, and ACE inhibitors post-MI) became routine. Surgical fields, traditionally more experience-based, also embraced evidence: trials in the 2000s comparing surgical techniques or demonstrating the benefit of perioperative beta-blockade, for instance, informed practice. Professional societies started guideline committees that follow rigorous processes. By the early 1990s, groups like the American Urological Association had pioneered formal guideline development methods, requiring systematic literature searches and explicit rating of evidence quality.
- Nursing and Allied Health: The principles evolved into what is often termed Evidence-Based Practice (EBP), encompassing nursing, physiotherapy, occupational therapy, and other allied fields. These disciplines adopted EBP to ensure that non-physician healthcare providers also base their interventions (from wound care protocols to rehabilitation exercises) on solid evidence. Nursing, for instance, developed evidence-based protocols for infection control, patient positioning to prevent pressure sores, etc. The spread of EBP beyond medicine – to education, public health, management – was facilitated by the general appeal of making decisions based on systematically collected evidence rather than tradition.
- Public Health and Policy: We will discuss health policy more in Chapter 5, but it’s notable that even in public health (e.g., recommendations for screenings or vaccinations) the concept of evidence grading and systematic reviews (like the Community Guide in the US, or WHO’s evidence-based guidelines) became standard.
In medical education, EBM has become a staple. Medical students now learn how to read papers critically; residents partake in journal clubs and are often required to frame clinical questions and search for evidence as part of their training. Many programs have a dedicated EBM curriculum or rotations. This represents a profound change from prior generations of trainees who learned mostly through apprenticeship; now they are explicitly taught how to continuously update their knowledge using scientific literature. By emphasizing lifelong learning skills, EBM has helped clinicians cope with the ever-expanding medical literature. As one illustration of the need, by the mid-2000s thousands of articles were being published each year across journals – no practitioner could read them all, but an evidence-based approach helps identify and focus on the high-quality, relevant research.
EBM also encouraged the development of information tools to support practice. Synopsis journals (publishing one-page distilled appraisals of new studies), online clinical query services, and evidence-based point-of-care tools have their roots in the 1990s EBM movement’s recognition of the information overload problem. A 2005 audit famously found that managing a single day’s worth of acute medical admissions would require reading 3,679 pages of guidelines (estimated 122 hours of reading) to cover all the conditions – an impossible task for any individual. The response has been to create summaries, filters, and synopses to make evidence more digestible and accessible at the bedside.
In sum, the methodology and practice of EBM are now deeply ingrained across healthcare. Clinicians ask structured questions, seek high-level evidence, appraise studies for quality, and apply findings in a patient-centered manner. The approach has shown clear benefits: therapies with strong evidence have been widely adopted (improving outcomes), while those found to be ineffective or harmful have been abandoned. However, EBM is not without challenges in practice – time constraints, access to information, and variable skill in appraisal can be barriers. Chapters 4 and 5 will discuss both the positive impacts and some limitations and criticisms of EBM’s real-world application. First, we turn to how this evidence-based approach has concretely transformed clinical decision-making and patient care.
Chapter 4: Impact of EBM on Clinical Decision-Making and Patient Care
4.1 Transforming Clinical Decision-Making
The advent of evidence-based medicine has significantly reshaped the mindset and process of clinical decision-making. Traditionally, a physician’s decisions were guided largely by personal clinical experience and the teachings passed down by mentors and authoritative textbooks. EBM introduced a new question at the point of care: “What is the evidence that this intervention is effective and safe for my patient?” This seemingly simple question has led to more standardized and objective decision-making in many areas of medicine.
One clear impact is the reduction in inappropriate practice variation. As mentioned in Chapter 2, wide variations in how physicians managed similar conditions were documented in the pre-EBM era. With EBM’s emphasis on best practices derived from research, many aspects of care have become more uniform and aligned with evidence-based guidelines. For example, prior to EBM, management of a condition like unstable angina could vary widely between hospitals or physicians; now, there are well-established protocols (such as giving antiplatelet therapy, statins, heparin, etc.) based on major clinical trials, and adherence to these protocols is considered a quality benchmark. This kind of standardization based on evidence tends to improve outcomes, because patients are more likely to receive effective interventions and less likely to receive ineffective or harmful ones.
Clinicians have become more critical and reflective in their decision-making. EBM taught a generation of doctors to question the status quo. Rather than “We do this because that’s how I was trained” or “because it’s tradition,” the culture shifted towards “Is there evidence to support doing this?” For instance, routine use of certain therapies (like prescribing antibiotics for viral infections or using certain types of hormone therapy) has been curtailed due to evidence showing lack of benefit or even harm. This critical approach is supported by tools like clinical practice guidelines and decision aids that distill evidence into actionable choices.
Shared decision-making has also been bolstered by EBM. When evidence is available about the benefits and risks of options, clinicians can more effectively communicate these to patients and involve them in decisions. Take the example of localized prostate cancer: there are options ranging from active surveillance to surgery to radiation, each with different profiles of potential benefit and side effects. EBM provides data on outcomes (e.g. survival rates, risk of incontinence or impotence), enabling a more informed discussion with the patient about what choice aligns with their preferences. Without evidence, such discussions would be speculative; with evidence, they can be concrete (e.g., “Radiation and surgery have similar 10-year survival in studies, but surgery has a slightly higher risk of long-term incontinence; how do you feel about these trade-offs?”).
It’s important to note that clinical decision-making is still an art as well as a science. EBM doesn’t turn clinicians into automatons following guidelines blindly – rather, it equips them with information. The clinician must still interpret and apply that information judiciously. In scenarios where a patient doesn’t neatly fit the profile studied in trials (e.g., multiple co-morbidities, extremes of age, etc.), the physician must decide whether evidence can be extrapolated or if an individualized approach is needed. EBM provides a framework to do so transparently, acknowledging uncertainty when evidence is lacking. In fact, one of the salutary effects of EBM has been to highlight where evidence is lacking, prompting either caution in decisions or further research. A clinician might say, “The evidence for treating patients over 90 years old with this drug is scant, but given what we know in younger patients and considering your health goals, I suggest we still try it cautiously.” This kind of reasoning – explicitly considering evidence and its limits – was not routine before EBM.
Another impact of EBM on decision-making is the proliferation of clinical decision support tools. Many electronic health record (EHR) systems now incorporate alerts or suggestions based on evidence-based guidelines (for example, an alert if a patient with heart failure is not on an ACE inhibitor, since strong evidence shows it improves survival in eligible patients). These tools are direct descendants of the EBM movement, translating evidence into practice prompts. They remind or guide clinicians at the point of care according to established evidence. While sometimes viewed as intrusive, when well-implemented they can improve adherence to evidence-based practices, especially for things easily overlooked.
However, the influence of EBM on decision-making is not uniformly positive in everyone’s eyes. Some critics have pointed out that an overly rigid adherence to guidelines or algorithms can oversimplify or dehumanize care. For instance, a guideline might recommend a particular medication as first-line therapy for most people, but a thoughtful clinician might identify reasons why a particular patient should deviate from that (perhaps due to co-morbid conditions, or a past bad experience with a similar drug). There have been concerns about “cookie-cutter” medicine – that doctors might abdicate thinking in favor of just following algorithms. EBM proponents counter that guidelines are guides, not shackles, and that real EBM means applying general evidence to the individual case, not ignoring individual differences. The ideal is a balanced decision-making process: informed by evidence but tailored by clinical judgment and patient input.
In practice, many studies have documented improvements in patient outcomes attributable to evidence-based interventions. For example, mortality after myocardial infarction dropped significantly from the 1980s to 2000s in large part because of the widespread adoption of evidence-based therapies (thrombolysis or primary angioplasty, beta-blockers, ACE inhibitors, statins, etc.). Similar trends are seen in HIV (where evidence-based combination antiretroviral therapy turned a fatal illness into a chronic condition) and many cancers (where evidence from clinical trials has steadily improved cure or survival rates). While not every improvement in medicine can be directly tied to EBM (technological advances and other factors play roles), it’s clear that systematically testing interventions and then implementing those that work has saved and improved lives.
4.2 Improvements in Patient Care and Outcomes
EBM’s ultimate goal is better patient care – measured in outcomes like longer survival, symptom reduction, improved quality of life, and fewer adverse events. By emphasizing treatments proven to work and cautioning against those proven ineffective or harmful, EBM has helped to improve the overall quality of care. For example:
- The use of aspirin, beta-blockers, and statins in patients at high risk of cardiovascular events is now standard and has prevented countless heart attacks and strokes, thanks to solid evidence.
- Many surgical procedures that were common in the past (e.g., routine tonsillectomies in children, certain types of knee surgeries for osteoarthritis) have been reduced after evidence showed they provided minimal benefit over conservative management. This spares patients unnecessary operations and the associated risks.
- Conversely, some interventions that were underutilized got a boost. One example is the use of corticosteroids in preterm labor to help fetal lung maturity – an idea proven in trials in the 1970s but not widely adopted until systematic reviews in the 1990s (including a famous early meta-analysis) convinced obstetricians of its efficacy. This evidence-based change greatly improved neonatal outcomes for preterm infants.
Patients today are more likely to receive treatments that have been validated in rigorous studies. Clinical practice guidelines – often developed by panels of experts systematically reviewing evidence – exist for hundreds of conditions. These guidelines give clinicians checklists of evidence-based care processes (for instance, the care of trauma patients now follows evidence-based protocols like ATLS, and sepsis management follows evidence-based bundles of care). Adherence to such guidelines has been linked to improved survival and fewer complications in many studies. For example, in pneumonia, timely antibiotics and appropriate selection (as guided by evidence) improve outcomes; in stroke, evidence-based use of thrombolysis and organized stroke unit care improves recovery. EBM has essentially created a more scientific, outcomes-focused approach to each disease.
The focus on patient outcomes has also led to identifying and discontinuing ineffective or harmful practices, which is just as important for patient welfare. A tragic example is the use of anti-arrhythmic drugs post-heart attack: for years, these were used because they suppressed arrhythmias (a plausible benefit), but evidence (the CAST trial in 1989) showed they actually increased mortality. Thanks to EBM, such drugs were largely abandoned for that indication, likely saving lives. Another example: hormone replacement therapy (HRT) in postmenopausal women was widely touted to prevent heart disease based on observational data, but the Women’s Health Initiative (an RCT) found that certain HRT regimens actually increased cardiovascular risk and breast cancer risk, leading to a dramatic change in practice around 2003. These examples illustrate EBM’s corrective function – ensuring patient care is not only about doing more, but doing what truly helps. The ongoing “Choosing Wisely” initiative, which started in the 2010s, is an example of evidence-based recommendations to reduce overtreatment (identifying tests or treatments that evidence indicates are unnecessary). It has roots in EBM’s insistence on value and effectiveness.
Patients have also benefited from improvements in safety and quality of care due to evidence-based protocols. Infection rates in hospitals have been reduced by following evidence-based infection control practices (like hand hygiene, sterile technique for central line insertion – which came from evidence showing huge reductions in line infections). The safety of surgery improved with evidence-based checklists (the WHO Surgical Safety Checklist is evidence-backed and has reduced surgical mortality). These practical changes often stem from aggregating evidence and best practices, demonstrating that EBM is not just about drugs and devices, but also about process improvements.
Despite these advances, there is recognition that there remains a gap between evidence and practice. Classic studies have shown that a significant proportion of patients do not receive recommended evidence-based care. For example, a well-known study in the early 2000s found that on average, only about 55% of recommended care (based on evidence-backed quality indicators) was actually delivered to patients in the U.S.. In other words, about half the time, care did not follow what the evidence and guidelines would suggest. This gap has multiple causes: lack of awareness or familiarity with evidence by providers, system constraints, patient factors, etc. Reducing this gap is a major goal of ongoing quality improvement efforts and speaks to the need for better implementation of EBM (sometimes called the field of implementation science). Nonetheless, even with imperfect implementation, the trend has been toward more evidence-concordant care over time.
4.3 Patient-Centered Care and EBM
An important aspect of EBM’s impact on patient care is how it dovetails with patient-centered care. Initially, EBM was criticized by some as being too population-focused or “one-size-fits-all,” potentially clashing with individualized care. However, over time, it has been increasingly emphasized that EBM and patient-centered care are complementary. Using evidence is part of providing the best care, but it must be balanced with the individual’s needs and circumstances.
In practice, this means clinicians use evidence to inform patients about their options in an understandable way. Decision aids (pamphlets, videos, or interactive tools) have been created for many conditions (like options for early-stage breast cancer treatment, or for whether to undergo prostate cancer screening). These aids are evidence-based, presenting the potential outcomes and probabilities in plain language. Studies have shown that when patients are better informed of the evidence, they often make choices more aligned with their values – for example, some may choose less invasive management if they understand that aggressive treatment offers minimal extra benefit. This contributes to higher patient satisfaction, and often to care that isn’t just evidence-based but also values-based.
EBM’s influence has also reached the realm of health literacy and patient empowerment. Patients today have more access to medical information (via the internet, etc.), and many come to consultations armed with knowledge from sources like Cochrane summaries or clinical trial reports they have read. While information quality varies, the general trend is that patients expect evidence-based answers from their doctors. An informed patient might ask, “Is this treatment really necessary? What does the research say about its benefits and risks?” Clinicians comfortable with EBM are better equipped to have those discussions. This can improve trust, as the physician isn’t just saying “Because I said so,” but “Here’s what the research shows, and here’s how it applies to you”.
In certain fields, such as oncology, using evidence to guide and explain choices is now standard. Oncology has become highly evidence-driven, with treatment protocols (often detailed in NCCN or ESMO guidelines) that outline exactly what the recommended options are at each stage of disease, based on trial evidence. Oncologists frequently discuss clinical trial data (survival percentages, side effect profiles) with patients when choosing regimens. This approach ensures patients have realistic expectations and a clear rationale for their treatment plan.
However, not all effects on patient care have been positive or straightforward. There is an ongoing discussion about the unintended consequences of the evidence-focused era. For example, some point out that the focus on metrics and guidelines can lead to “treating the numbers” rather than the patient – e.g. aggressively trying to hit a guideline-recommended blood pressure or blood sugar target without sufficient regard to the patient’s frailty or preferences (a scenario sometimes referred to as the tyranny of metrics). Others note that with so much information and guidelines, there is a risk of information overload for both clinicians and patients. Patients might be overwhelmed if presented with too many statistics or options, which requires clinicians to be skilled communicators in conveying evidence without causing confusion or undue anxiety.
Another debated issue is how well EBM accounts for complex patients, such as those with multiple chronic conditions. Clinical trials often focus on one condition in isolation, and guidelines typically address one disease at a time, whereas many real patients have several diseases simultaneously. Applying multiple single-disease guidelines to one patient can result in a burdensome regimen (for instance, a patient with diabetes, heart disease, and arthritis might end up on 10 medications if each guideline is followed to the letter). Here, good clinical judgment and patient input must reconcile evidence with practicality and quality of life. The EBM community is aware of this and has been exploring ways to produce more patient-centered guidelines or approaches (like guidelines for multi-morbidity). It’s part of the maturation of EBM to handle these nuances.
Finally, EBM has had a role in shaping health expectations: both doctors and patients now expect proof that interventions work. The culture of medicine has become one where introducing a new drug or procedure almost always requires evidence from clinical trials. Patients might even ask, “Is this treatment experimental or is it proven?” That expectation is a positive development for patient safety and efficacy, though it can sometimes slow adoption of innovations (a necessary trade-off to avoid harm). The COVID-19 pandemic, for example, illustrated EBM’s influence: there was intense demand for evidence from trials for various treatments (remdesivir, dexamethasone, monoclonal antibodies, vaccines), and practices shifted rapidly when high-quality evidence became available, demonstrating the system’s responsiveness to evidence.
In conclusion for this chapter, EBM has deeply impacted clinical decision-making and patient care for the better, making medicine more data-driven, transparent, and consistent. Patients generally receive more effective care and are increasingly part of the decision process. Yet, challenges in implementation, individualization, and information management remain. The next chapter will extend this discussion to the macro level: how EBM has influenced health policy, guidelines, and the organization of healthcare beyond individual patient encounters.
Chapter 5: Influence of EBM on Health Policy and Health Systems
5.1 Evidence-Based Guidelines and Standards of Care
One of EBM’s most significant contributions to health policy is the development of formal clinical practice guidelines and standards of care that inform healthcare delivery on a broad scale. Prior to the 1990s, guidelines (if they existed) were often consensus statements by experts without a systematic evidence review. EBM introduced rigor into guideline development. Organizations began assembling panels that included content experts, methodologists, and sometimes patient representatives, who would perform systematic literature reviews and then formulate recommendations graded by strength of evidence. By the late 1980s and early 1990s, multiple medical professional bodies had started this process. For example, the American Heart Association and American College of Cardiology issued evidence-based guidelines on cardiac life support and cholesterol management; the American Diabetes Association began yearly updates of evidence-based standards for diabetes care.
Health policy-making bodies also embraced this approach. The US Agency for Health Care Policy and Research (AHCPR, now AHRQ) in the early 90s sponsored guideline development for common topics like low back pain and depression, emphasizing evidence review. Internationally, countries established agencies (e.g., Canada’s CTFPHC, UK’s NICE a bit later in 1999) that took evidence synthesis as the basis for recommendations. NICE (National Institute for Health and Care Excellence) in the UK is a prime example: it was set up to provide evidence-based guidance not just on clinical treatments but also on public health interventions and even cost-effectiveness. NICE’s technology appraisals became a model for using evidence (including economic evidence) to inform what interventions should be available in the National Health Service.
These guidelines and recommendations have powerfully influenced practice by defining what is the “standard of care.” They serve as references for clinicians (“If I follow the guideline, I’m likely giving appropriate care”) and also for payers and regulators. For instance, many insurance companies or national health services tie reimbursement or coverage decisions to guideline-endorsed therapies. In the United States, the Affordable Care Act (2010) even linked certain policies to evidence-based guidelines – e.g., it mandated that preventive services with strong evidence (graded A or B by the US Preventive Services Task Force, USPSTF) must be covered by insurers without patient co-pay. This is a direct translation of evidence into policy: if high-level evidence shows a preventive measure saves lives or health (like colonoscopy for colon cancer screening, or immunizations), policy ensures it’s made accessible.
Another area is quality measurement and improvement programs that rely on evidence-based benchmarks. The rise of pay-for-performance (P4P) schemes in the 2000s (in Medicare and various health systems globally) was predicated on the idea that we can measure adherence to evidence-based processes and link them to incentives. For example, a P4P program might reward hospitals that ensure heart attack patients are discharged on all the evidence-based medications (aspirin, beta-blocker, statin, ACE inhibitor). The assumption, borne out by evidence, is that those processes correlate with better patient outcomes. The use of these programs reflects policy-makers’ acceptance that evidence-based care is better care, and their willingness to push the system towards it through financial levers.
5.2 Health Technology Assessment and Resource Allocation
EBM has also deeply impacted how new medical technologies (drugs, devices, procedures) are evaluated and adopted through health technology assessment (HTA) processes. In many countries, governments or expert panels systematically review the clinical evidence (and often cost-effectiveness data) for new interventions to decide if they should be approved, covered by insurance, or recommended for use. This can be seen as a macro-level EBM: not just a doctor deciding what’s best for a patient, but a health system deciding what’s best for a population, based on evidence.
For instance, agencies like the U.S. Preventive Services Task Force (USPSTF) evaluate preventive interventions (screenings, counseling) with strict evidence criteria and assign grades that influence public health policy and insurance coverage. The World Health Organization leverages evidence-based processes to update its Essential Medicines List and issue guidelines on public health issues (like the optimal management of tuberculosis or HIV, or responses to pandemics). The Cochrane Collaboration’s work feeds directly into many of these decisions – Cochrane Reviews have informed numerous WHO guidelines and national policies by providing high-quality syntheses of evidence.
In the context of pharmaceuticals, regulatory bodies such as the FDA (Food and Drug Administration) in the US or EMA (European Medicines Agency) in Europe have long required evidence of efficacy and safety (through clinical trials) for drug approval – that predates EBM. However, what EBM added is the expectation of comparative effectiveness and cost-effectiveness in many cases. For example, Europe and Canada often require or strongly consider not just whether a drug works better than placebo, but how it compares to existing alternatives and whether its benefits justify its cost. This has led to some high-cost drugs being restricted or negotiated down in price if evidence showed only marginal improvements. Insurance coverage decisions in many systems involve expert panels that review evidence (often including systematic reviews) to decide if a therapy is “medically necessary and evidence-based.”
EBM’s influence is also evident in coverage policies and benefit design. Insurers have, for better or worse, taken up the language of evidence: treatments deemed experimental or without sufficient evidence may not be covered, whereas those strongly supported by evidence usually are. While cost considerations can muddy the waters, it is common now for payers to require evidence such as randomized trial data before approving expensive interventions. Some countries explicitly use EBM principles to decide on funding – e.g., the UK’s NICE will not endorse an intervention unless evidence shows a favorable cost per quality-adjusted life year (QALY) below a certain threshold, ensuring that limited resources go to interventions with solid evidence of benefit.
On a broader scale, evidence-based health policy attempts to apply the same rigor to public health interventions and system design. For instance, policymakers might use evidence from community trials or observational studies to craft policies on tobacco control, dietary guidelines, or trauma system organization. In the last two decades, terms like “evidence-based public health” or “evidence-based policy” have gained traction. They argue that just as clinicians should use evidence, so should policymakers when deciding things like: Should we implement a soda tax? Does providing housing for homeless individuals improve health outcomes enough to justify the investment? These complex decisions are not purely medical and involve social values and feasibility, but the EBM ethos encourages using the best available data rather than guesses.
It must be acknowledged, however, that policy decisions are not made on evidence alone – economics, ethics, and political factors play roles. EBM has given policymakers better tools to know what works (e.g., which interventions reduce disease burden), but whether and how to implement those can depend on budgets and public acceptability. There is also a recognized difference between evidence-based medicine and evidence-based policymaking: the latter requires considering context, stakeholder values, and sometimes acting on imperfect evidence. Some critics have pointed out that policy often lags behind evidence – for example, despite evidence of harm, it took a long time for many healthcare systems to de-adopt the routine use of certain tests or treatments due to inertia or vested interests. Conversely, sometimes policy runs ahead of evidence, driven by urgency or public pressure (as seen in some decisions during health crises when evidence is still emerging).
Despite these nuances, the overall trend is that evidence is now a key currency in health policy debates. Advocates for a policy will bring systematic review data to support their case; health authorities justify guidelines and coverage with reference to trial data; and global bodies set goals (like the UN’s health-related Sustainable Development Goals) partly based on evidence of what interventions can achieve. The language of evidence – systematic reviews, meta-analyses, statistical significance, etc. – has moved from journals into policy documents and even legislation.
5.3 Quality and Accountability in an Evidence-Based Era
Another facet of EBM’s influence on health systems is the push for accountability and quality measurement. If evidence defines what should be done, then measuring whether it is being done becomes possible and indeed expected. Healthcare systems now regularly collect data on performance indicators, many of which derive from evidence-based guidelines. For example, hospitals track infection rates, readmission rates, surgical complication rates, etc., because evidence suggests these reflect quality of care and can be improved by following best practices. Public reporting of such metrics (e.g., publishing hospital infection rates or cardiac surgery outcomes) is increasingly common, under the premise that transparency and accountability will drive improvement. This is an extension of EBM: shining a light on whether evidence-based care is delivered, and making it part of institutional expectations.
In many countries, accreditation bodies (like Joint Commission in the U.S.) and payers have instituted “core measures” for hospitals that are explicitly evidence-based. One widely cited example was a study in 2003 (McGlynn et al., NEJM) that found adults in the U.S. received only about 55% of recommended care for preventive, acute, and chronic conditions. This eye-opening result spurred efforts to close the gap. Through the 2010s, improvements were documented in several areas thanks to concerted quality initiatives – for instance, far more heart attack patients began receiving all guideline-indicated therapies before discharge, and as a result, 30-day survival improved.
Pay-for-performance and value-based care models, as touched on, tie financial incentives to evidence-based care delivery. Medicare’s Value-Based Purchasing and similar programs in other countries essentially monetize EBM: if you deliver evidence-supported care and achieve good outcomes, you receive bonuses; if not, you might face penalties. The evidence behind these programs is mixed – some studies show modest improvements, others show providers gaming metrics or neglecting unmeasured aspects of care. Nonetheless, they underscore that EBM has permeated even the business side of healthcare.
From a patient perspective, the benefit of all this is that when you go to a hospital or clinic in an evidence-driven health system, you are more likely now than 30 years ago to receive care that is aligned with the latest knowledge. A patient with a stroke will likely be treated in a stroke unit with protocols proven to improve outcomes, a patient with cancer will get treatment per evidence-based pathways with known efficacy rates, and a child will receive immunizations and preventive care per evidence-based schedules (assuming access is not a barrier). There are still disparities and areas of low-value care, but the baseline standard has risen.
Policy challenges and controversies: EBM’s application in policy hasn’t been without contention. Sometimes, strict evidence-based recommendations face pushback from professionals or the public. One famous example is mammography screening guidelines: when the USPSTF updated evidence and suggested less frequent screening for certain age groups based on a balance of benefits and harms, it sparked public and political backlash that led to policy overriding the recommendation. This shows that even if evidence suggests a course, societal values and fear can lead to a different decision – a reminder that evidence-based policy must also consider communication and public trust.
Another area of debate is “rationing” vs. “wise use of resources.” EBM provides a rationale for not covering or paying for interventions that don’t work or are inferior. While this seems straightforward, there are instances where patients or clinicians strongly believe in something despite lack of evidence (or presence of negative evidence). For example, certain experimental therapies or supplements might be demanded by patients. An evidence-based approach might deny those under public funding, which can lead to accusations of rationing. Health systems have to navigate these issues carefully, emphasizing that denying an ineffective treatment isn’t rationing – it’s avoiding waste and potential harm – but such decisions are often sensitive.
Finally, the EBM movement has stimulated the creation of global collaborations and databases for evidence sharing. Initiatives like the GRADE Working Group (for consistent evidence grading) and the Guidelines International Network (G-I-N) foster international standards for developing and reporting evidence-based guidelines. The idea is to avoid each country duplicating work and to learn from each other’s evidence evaluations. This global perspective is especially useful when dealing with diseases that cross borders (like pandemics, where pooling evidence quickly is vital).
In summary, EBM has extended its reach from the bedside to the boardroom, so to speak. It has provided the intellectual framework for making healthcare more systematic, justifiable, and transparent at all levels. Health policies now routinely invoke evidence as the basis for action (or inaction), and health systems strive to implement what research has shown to be effective. While challenges of implementation, equity, and maintaining clinician autonomy remain, the net effect has been a move toward more rational, effective healthcare systems. With this understanding of the current state of EBM’s impact, we now turn to the future: how emerging technologies, particularly artificial intelligence, are poised to influence and possibly transform evidence-based medicine in the coming years.
Chapter 6: The Future is Now – Artificial Intelligence in Evidence-Based Medicine
The 21st century has seen an exponential growth in data relevant to healthcare: electronic health records, genomic data, vast research outputs, real-time patient monitoring, and more. This deluge of information presents both an opportunity and a challenge for evidence-based medicine. Artificial Intelligence (AI) – particularly advanced machine learning algorithms – offers tools to analyze and leverage these data in ways humans cannot easily do unaided. In recent years, AI has begun to intersect with EBM, promising to enhance how we generate evidence, how we synthesize it, and how we apply it at the bedside. This chapter explores current and emerging applications of AI in the context of EBM, spanning predictive modeling, evidence synthesis (e.g., systematic reviews), and clinical decision support systems. It also considers how AI can enable a more continuous and responsive evidence-based practice, sometimes described as “EBM 2.0”.
6.1 AI and Predictive Modeling in Clinical Medicine
Predictive modeling in medicine involves estimating the likelihood of future outcomes (like disease occurrence, disease progression, or treatment response) based on patient data. Traditional EBM uses relatively simple models or risk scores – for example, the Framingham Risk Score for predicting cardiovascular events or the APACHE score in ICU for mortality risk. These were derived using statistical methods on cohort data, essentially early forms of data-driven evidence. AI, especially modern machine learning, can take predictive modeling to a new level by handling far more complex patterns and larger datasets than classical statistical methods.
Machine learning algorithms (like neural networks, random forests, gradient boosting machines) can analyze high-dimensional data – such as thousands of gene expressions, imaging pixels, or detailed EHR records – to find patterns associated with outcomes. For example:
- In radiology, AI image analysis has achieved expert-level performance in detecting pathologies such as lung nodules on CT scans or diabetic retinopathy on retinal photographs. These AI models are trained on large image datasets with known diagnoses and learn to predict the probability of disease. Integrating this into practice means an evidence-based approach where AI provides a reading or a second opinion to assist radiologists, potentially catching findings that humans miss or speeding up interpretation.
- In oncology, AI models are being developed to predict tumor behavior or treatment responses based on histopathology slides (so-called “digital pathology”) and genomic profiles. This could inform evidence-based personalized treatment plans – for instance, an AI might predict that a certain patient’s cancer is likely resistant to standard chemotherapy but susceptible to a targeted drug, which could generate a new form of evidence (albeit requiring validation) to guide therapy.
- In primary care or hospital medicine, predictive algorithms analyze EHR data to identify patients at high risk for outcomes like sepsis, readmission, or complications. Some hospitals have deployed AI-based early warning systems that can flag, say, a patient who might deteriorate in the next 24 hours by continuously analyzing vital signs, lab results, and notes. This predictive alert can be seen as an evidence-based tool if it’s derived from training on outcomes data – effectively encapsulating evidence from many past patient trajectories to inform current care.
From an EBM perspective, AI-driven predictions must be rigorously validated. Just as a new diagnostic test or risk score would need evidence of accuracy and clinical utility, AI models need prospective studies to confirm that their use actually improves patient outcomes or decision-making. Encouragingly, some such studies are emerging. For instance, there have been trials of AI-guided care, like an AI-based decision support for managing blood pressure or blood glucose, showing improvements in those parameters compared to usual care.
One domain that merges AI, prediction, and EBM is clinical prognostic modeling. Traditional prognostic models often use logistic regression based on a handful of variables. AI can incorporate a much wider range of data (e.g., thousands of features from labs, imaging, genomic, and even patient social data) to potentially yield more accurate or individualized predictions. For example, the “predictive analytics” used in some health systems might identify a patient with heart failure at rising risk of hospitalization and prompt proactive interventions. This is evidence-based in the sense that it’s drawing on patterns learned from evidence (prior data), and it could lead to evidence generation if we test it in a trial.
However, AI predictions also challenge EBM’s traditional evidence hierarchy. Many AI models are developed on real-world observational data, not RCTs. If an AI model predicts an outcome with high accuracy, is that enough evidence to change practice, or do we need RCTs of using the model? The likely answer is that for AI to change clinical decisions, we want evidence that acting on the model’s predictions helps – which often means an interventional trial. For instance, one could test: does an AI sepsis alert system, when implemented, reduce mortality compared to no AI system? If yes, that evidence would support adoption. This process is ongoing, and until proven, such AI tools are often used in a supportive role.
The synergy of AI and EBM in prediction might eventually allow a more personalized evidence-based medicine. Classic EBM tends to apply population-derived evidence to the average patient; AI can potentially stratify patients into narrower categories. For example, rather than saying “Drug X has a 50% success rate in clinical trials,” an AI could analyze an individual’s profile and say “Given this patient’s specific characteristics, the success probability is 80%” (or 20%, as the case may be). This level of personalization, if accurate, would be transformative. It’s akin to having an evidence-based guideline for each unique patient. We are not fully there yet, but research into AI-guided therapy choices in fields like oncology and cardiology is accelerating.
In summary, AI predictive modeling is expanding the toolkit of EBM by adding sophisticated ways to interpret evidence from large datasets for individual care. It complements evidence from trials with evidence from big data. Yet, to maintain EBM principles, these models must undergo scrutiny – validating their predictions and ensuring they lead to better patient-centered outcomes, not just impressive accuracy metrics.
6.2 AI in Evidence Synthesis: Automating Systematic Reviews
Systematic reviews and meta-analyses are bedrocks of EBM, but they are labor-intensive and time-consuming. A high-quality Cochrane review can take many months (or even years) from conception to publication, involving exhaustive literature searches, screening of potentially thousands of abstracts, data extraction from included studies, and qualitative or quantitative synthesis. The volume of research output has been growing so fast that keeping reviews up-to-date is a perpetual challenge – by the time a review is published, new studies may already be out. Artificial intelligence offers solutions to expedite and even partly automate the evidence synthesis process.
Several areas of the systematic review pipeline have seen AI applications:
- Literature Searching and Screening: One of the first and most successful uses of AI in reviews has been in citation screening. Machine learning models (often using text classification algorithms) can be trained to distinguish relevant studies from irrelevant ones based on an initial set of included and excluded references. For example, after a human reviewer screens a few hundred abstracts (labeling them include/exclude), an AI model can learn that pattern and help prioritize the remaining thousands, effectively reducing the workload by filtering out obviously irrelevant papers and highlighting probable inclusions. Tools like the open-source ASReview (Active Semi-supervised Review) use active learning to interactively train on reviewer decisions and have shown ability to substantially cut down screening burden while missing few if any relevant studies. Cochrane and other organizations are already using machine learning classifiers to assist with this step, making the process faster.
- Data Extraction and Risk-of-Bias Assessment: AI, particularly natural language processing (NLP), is being developed to auto-extract key information from trial reports, such as sample sizes, outcomes, effect sizes, etc. Early systems (like MIT’s RobotReviewer) have demonstrated the capability to read trial publications and output a draft risk-of-bias assessment. For instance, it might flag phrases in a paper indicating whether allocation was randomized or blinded. While not yet at the point of completely replacing human judgment, these tools can save time by pre-populating evidence tables or highlighting relevant text for reviewers to verify. As NLP models (especially large language models) improve, we might see them summarizing entire study results or combining data across studies automatically.
- Living Systematic Reviews: AI is particularly suited to the concept of “living” systematic reviews – reviews that are continually updated as new evidence emerges. With automated search alerts and AI screening, a pipeline can be set up where new publications are fed into the system, and the AI determines if they are relevant and, if so, incorporates their data into the existing review structure. Some review teams have started doing this, using semi-automation to maintain up-to-date evidence for rapidly evolving topics (an example was living reviews during the COVID-19 pandemic where evidence was changing weekly). AI makes the update process more feasible by handling much of the repetitive work.
- Evidence Mapping and Gap Detection: Beyond traditional reviews, AI can quickly map broad swathes of literature. For example, topic modeling or clustering algorithms can analyze thousands of abstracts to identify what subtopics or questions have been studied and where evidence might be thin. This helps researchers and funders identify research gaps – something Evidence-Based Medicine values so that new studies target unanswered questions rather than duplicating known results. As noted in one analysis, AI could help “detect research gaps and avoid funding redundant studies”.
- Summarization: With advanced language models, there’s now experimentation with having AI write parts of the review text. An AI could potentially draft a plain language summary of findings, or even the first pass of the discussion section by collating the key points from included studies. While human reviewers must supervise and edit such outputs (to ensure accuracy and prevent issues like AI hallucinations or misinterpretations), it can accelerate the authoring process.
The integration of AI in systematic reviews aligns with maintaining rigor if done carefully. For instance, Cochrane has established an “Evidence Pipeline” initiative, where machine learning helps sift through the torrent of new trial reports so human experts can focus attention efficiently. They’re also working on policies for the responsible use of such AI, acknowledging that transparency is crucial – e.g., if an AI-assisted method was used, it should be reported and ideally open to scrutiny.
One real-world impact is that with AI assistance, systematic reviews could become more timely and responsive, reducing the lag between evidence generation and evidence synthesis. Traditionally, evidence might take years to percolate into guidelines (sometimes cited as 5-10 years lag). With more agile reviewing processes, that could shrink. This is important in fast-moving fields or public health emergencies.
Moreover, if systematic reviews – the pinnacle of evidence – can be kept up to date more readily, then EBM as practiced by clinicians will be working off the latest information. Imagine a future where a clinician query (“what’s the evidence for treatment X in condition Y?”) is answered by an AI that has essentially done a real-time meta-analysis of current literature. That’s a conceivable extension of current technologies, essentially automating an EBM literature review on-the-fly.
However, challenges remain. AI models can have biases – e.g., a screening model might inadvertently learn to favor studies with certain keywords, potentially missing novel research that uses different terminology. Therefore, validation and human oversight remain paramount. The goal is not to remove humans from the loop, but to allow them to focus on tasks requiring judgment (like interpreting findings and making contextual conclusions) while AI handles more mechanical tasks (sifting, extracting, calculating). This human-AI partnership could greatly increase the throughput of high-quality evidence synthesis.
In summary, AI is helping to tackle the information overload that EBM faces. By accelerating systematic review workflows and making evidence synthesis more continuous, it addresses some current pitfalls of EBM (like slow updates and incomplete evidence integration). In doing so, AI is effectively becoming an enabler for the next generation of evidence-based medicine, where up-to-date evidence is readily at hand for decision-making.
6.3 AI-Powered Clinical Decision Support Systems
The pointy end of EBM is the clinical decision made for an individual patient. Traditionally, EBM informs this via guidelines, pocket references, or the clinician’s internalized knowledge from reading evidence. Now, AI-driven clinical decision support systems (CDSS) are emerging as tools to provide real-time, patient-specific recommendations at the bedside or in the clinic, essentially operationalizing EBM in software form.
AI-powered CDSS can take many forms:
- Diagnostic Support: Systems like IBM’s Watson for Health (and others) have been designed to ingest a patient’s symptoms, history, labs, even imaging, and propose possible diagnoses or clinical priorities. These systems often reference a knowledge base of medical literature. In an evidence-based framework, such a system might rank diagnoses not just by fit with symptoms but also by prevalence and known diagnostic probabilities (e.g., using Bayes’ theorem with evidence-based prior probabilities). Some simpler CDSS are already common – for instance, an alert if a patient’s presentation fits criteria for sepsis (based on evidence-based definitions and prognostic criteria). More advanced AI could integrate many data points (genetics, environment, etc.) to refine differential diagnoses. Importantly, AI can also help avoid missed diagnoses by comparing a case to thousands of past cases (something a single physician can’t do from memory). There have been cases where AI diagnostic tools caught rare diseases that clinicians initially overlooked, by pattern-matching to known presentations in the literature.
- Therapeutic Decision Support: AI can assist in choosing treatments by cross-referencing patient specifics with treatment guidelines and outcomes databases. For instance, in oncology, there are decision support systems that, given a cancer’s genetic markers, will suggest targeted therapies or clinical trial options, referencing evidence from trials of patients with similar tumor profiles. These systems essentially encapsulate large swathes of evidence (which no individual oncologist could memorize in full detail) and provide tailored suggestions. Another example is in cardiology – AI tools that calculate individualized benefit of interventions (like stenting vs. medication for coronary disease) based on a patient’s profile, gleaned from big datasets of similar patients and outcomes. This moves towards precision EBM, combining personal data with population evidence.
- Medication Management and Safety: Hospitals use AI to monitor prescriptions and flag potential issues. While simple drug-interaction alerts have existed for a while (based on known pharmacology), AI can do more: predict which patients are at risk of medication side effects by analyzing their records (who might develop opioid misuse, or whose kidney function is trending towards a level where drug dosing should be adjusted). It can also suggest optimal drug choices by comparing patient characteristics to trial inclusion criteria or known responders. For example, selecting an antidepressant might be a bit of trial-and-error; an AI might identify that a patient’s profile is similar to those who responded well to Drug A in past data, thus suggesting Drug A first, potentially improving the initial success rate.
- Workflow and Personalization: AI can tailor reminders and best-practice prompts to context. A blunt reminder might say, “Patients with diabetes need an eye exam annually.” An AI-enhanced one might prioritize or withhold that reminder based on context (perhaps it knows the patient already has an appointment, or conversely that this patient has missed several recommended steps and needs a special outreach). In this sense, it supports evidence-based practice (ensure guidelines are followed) but in a smarter, less intrusive way by reducing alert fatigue and focusing on gaps in care.
The integration of AI CDSS holds promise to make evidence-based recommendations more accessible. Instead of a physician needing to recall guidelines or search for evidence, the system can proactively bring relevant evidence to attention. For example, if a physician is ordering a test or drug, an AI CDSS might pop up: “Given the patient’s condition X, evidence-based guidelines recommend Y before Z. Do you want to consider that?” This kind of just-in-time information can improve adherence to evidence without the physician having to manually look it up.
AI can also help incorporate patient-specific factors into decisions in a way guidelines often don’t. Guidelines might say “first-line therapy for condition A is drug B,” but not account for, say, a patient’s comorbid condition C that makes drug B risky. An AI that has the patient’s full EHR can catch that and modify the recommendation (“Given comorbidity C, an equally effective alternative is drug D, which has evidence of safety in patients with C”). This cross-referencing of multiple conditions and treatments is something doctors do cognitively, but AI can help ensure nothing is overlooked, especially as patients accumulate many diagnoses and medications.
Another exciting frontier is conversational AI for decision support, such as advanced chatbot advisors. In the future, a doctor might query an AI assistant in natural language: “What does the latest evidence say about managing this patient’s rare condition given her specific circumstances?” and get a coherent summary. Already, large language models (like GPT-based systems) can summarize and explain evidence (though they need oversight to ensure accuracy). Cochrane and other EBM entities are investigating such use of generative AI – to answer PICO questions from their databases, for instance.
However, there are important considerations:
- Validation: Any AI CDSS must be validated in clinical trials or robust studies to ensure its recommendations improve outcomes and don’t lead to errors. A flashy AI is not automatically evidence-based; it must earn that status through evaluation. Some early uses of AI (like IBM Watson for Oncology) faced criticism when the AI’s recommendations did not always align with expert consensus or had flaws, partly because it wasn’t adequately tested in real practice settings.
- User Acceptance: Clinicians may be wary of black-box AI recommendations. EBM teaches skepticism and understanding evidence – so if an AI says “Do X,” clinicians will rightly ask, “Why? What’s the evidence or rationale?” This pushes developers towards explainable AI – providing human-readable justification for suggestions (e.g., “Recommendation based on patient’s profile matching cases in literature where treatment X led to 20% better survival”). Such transparency is crucial for trust and for training clinicians in a continuous EBM mindset.
- Integration with Workflow: A CDSS only helps if it fits seamlessly. Too many alerts or complex interfaces can cause alert fatigue or be ignored. The best systems operate in the background and surface only when there’s a significant evidence-based deviation or opportunity. For instance, an AI might silently check all hospitalized patients against evidence-based prophylaxis guidelines and only alert when it finds someone who appears to be missed (like a patient with high VTE risk not on prophylaxis, which evidence strongly recommends should be corrected). By intelligently filtering, AI can make evidence-based guidance more palatable and effective.
- Adaptation and Learning: A powerful notion is that AI systems can continuously learn from new data, effectively keeping themselves (and thus their advice) up-to-date. This is tricky in medicine because it would require ongoing validation and preventing drift into unsafe recommendations. But if done carefully, an AI CDSS could incorporate the latest evidence (e.g., the moment a new trial is published, it updates its knowledge base) faster than waiting for new guidelines to be written. In essence, the CDSS becomes a living embodiment of current evidence.
In summary, AI-powered decision support is an extension of the original vision of EBM: giving the right information to the right person at the right time to make the best decision. It can handle more data and more complex guidelines than a human can keep in their head, acting as a safety net and an advisor that ensures evidence – both general and personalized – is considered in every decision. Many of these systems are in pilot or development phases, but some have already shown benefits like reduced errors or better adherence to care standards. If carefully validated and implemented, they could become a standard part of clinical practice, much like how we now view hand-held devices or EHRs as standard tools.
6.4 Epistemological Shifts: From EBM to “EBM 2.0”?
The integration of AI into EBM is not just about doing the same tasks faster – it could represent a fundamental shift in how evidence is generated and used, a potential “EBM 2.0”. In traditional EBM, evidence flows one way: researchers produce it (through trials, etc.), then clinicians consume it via reviews and guidelines. In an AI-augmented world, the flow becomes more dynamic. Health systems using AI can become learning systems: every patient’s data can become part of the evidence (with appropriate privacy safeguards) through techniques like federated learning or large-scale observational analysis. AI can detect patterns in real-world practice – for instance, noticing that off-label use of a drug in some patients correlates with better outcomes, which might generate a hypothesis for a formal trial. The line between research and practice could blur, as AI continuously analyzes practice data to update evidence, and practice is continuously adjusted based on those findings.
This vision of a continuously learning healthcare system is sometimes called a “Learning Health System,” and AI is seen as a key enabler. It would mean that evidence-based medicine is always current and locally adapted: each health system might learn what works best for its population and feed that knowledge back (combined with global evidence) to improve care. It addresses a limitation of EBM: the evidence from trials may not always generalize to every context. With AI, local data becomes part of the evidence equation.
We see hints of this already in fields like critical care, where high-frequency data (heart rate, blood pressure waveforms) and interventions could be analyzed to find what patterns precede improvement or deterioration, then guiding interventions accordingly. If an AI learns that a certain sequence of ventilator adjustments often precedes improvement in ARDS patients, it can suggest that earlier next time – essentially deriving practice-based evidence.
In effect, AI could broaden the definition of “evidence” in EBM to include certain reliable outputs of algorithms analyzing big datasets (often termed “real-world evidence”). This challenges the classical hierarchy that placed RCTs at the top. We might see a parallel track of evidence: one from controlled experiments, another from AI-observed real-world patterns. The convergence or conflict of these will be a key issue for the future of EBM – which leads into the next chapter on epistemological implications.
For now, what’s clear is that AI is becoming an indispensable partner in managing the complexity of modern medicine. It promises to enhance EBM by tackling volume (lots of data), velocity (rapid insights), and variety (integrating different types of information). The result could be more accurate predictions, more efficient evidence reviews, and more personalized decision support – all aligning with the goal of better patient outcomes through evidence-based care. Yet, as the next chapter will discuss, this evolution must be guided carefully to maintain the scientific integrity and ethical grounding of medicine.
Chapter 7: Epistemological and Ethical Implications of Integrating AI into EBM
The melding of artificial intelligence with evidence-based medicine is not just a technological progression; it raises deeper questions about the nature of medical knowledge (epistemology) and about the ethical practice of medicine. In this chapter, we critically examine these implications. How does AI-generated insight fit into the hierarchy of evidence? Can a black-box algorithm be trusted in the same way as a randomized trial or a clinician’s judgment? What biases might AI introduce or perpetuate, and how do we safeguard against them? Who is accountable for decisions influenced by AI? These questions must be addressed to ensure that the integration of AI into EBM strengthens rather than undermines the quality and fairness of care.
7.1 Rethinking “Evidence”: Epistemological Challenges
EBM was built on a foundation of clinical epidemiology and biostatistics, valuing controlled experimentation and observation under clear protocols. The hierarchy of evidence (expert opinion < observational study < RCT < systematic review) implicitly values study designs where biases and confounders are minimized. AI, however, often derives its “knowledge” from pattern recognition in large datasets, many of which are observational or even purely correlational. This introduces an epistemological quandary: If an AI identifies a strong pattern (say, drug X is associated with better outcomes in subgroup Y), is that pattern to be considered evidence? Or must we confirm it with a traditional study?
Some argue that we may need to broaden our conception of evidence. Real-world evidence from big data, analyzed by AI, could supplement traditional trial evidence, especially in areas where RCTs are not feasible or timely. For instance, detecting rare side effects of drugs is often done through AI analysis of pharmacovigilance databases. That is considered evidence for regulatory action. Similarly, AI might reveal that a combination of factors predicts disease onset; it might not be a classical trial, but it’s valuable knowledge gained through inductive analysis. EBM will need to accommodate such evidence, possibly by developing frameworks to evaluate the quality of AI-derived insights (analogous to how we evaluate study quality).
However, the risk is confusing correlation with causation. AI can easily find spurious correlations in big data if not carefully constrained, leading to false “evidence”. For example, an AI might notice patients on a particular medication have better outcomes, but maybe that medication is given to generally healthier patients (confounding by indication). Traditional EBM tools like critical appraisal and causal inference need to be applied to AI outputs. This could mean requiring that any AI-discovered treatment effect either aligns with known biological plausibility or is tested in a prospective trial before being adopted widely.
The notion of a black-box algorithm making recommendations is at odds with the transparency valued in science. Typically, we trust evidence we can scrutinize – methods and data open to inspection. Many AI models, especially deep learning ones, are opaque; they don’t readily explain why they reached a conclusion. This raises an epistemic issue: should a physician trust an AI’s suggestion if they cannot understand its reasoning? Some scholars argue that if the AI’s performance (accuracy, outcomes) is proven, perhaps the lack of interpretability is acceptable – drawing analogies to trusting a well-validated diagnostic test without fully understanding its inner workings. Others push for explainable AI in medicine as a necessity, so that each recommendation comes with human-comprehensible justification, effectively converting the algorithm’s result into something resembling a piece of evidence a clinician can evaluate. For instance, rather than just saying “Recommended treatment: X,” an AI might add, “because in our database of 100,000 patients, those most similar to this patient had a 20% higher survival on X compared to Y.” That allows the clinician to gauge the strength of that evidence, including sample size and effect magnitude.
Another epistemological consideration is how AI might handle the interplay of the three pillars of EBM: evidence, clinician expertise, and patient preference. Traditional EBM sometimes struggled to integrate patient preferences systematically; AI could either exacerbate that (if it becomes a rigid oracle) or improve it (for example, some AI decision aids can elicit patient preferences via interactive chats and incorporate that into recommendations). The latter would align AI with the EBM principle that evidence alone is not enough – the patient’s context and values are crucial. But if AI systems are not carefully designed, there’s a risk they become overly directive, sidelining the individualized aspect of care.
Finally, consider the concept of continuous learning algorithms that update as they get more data (for instance, updating risk predictions as more outcomes are observed). This means the “evidence” an AI uses today might differ tomorrow – a moving target. How do we incorporate that into guidelines or malpractice standards? It challenges the static notion of a knowledge base. We might need a way to version or snapshot AI recommendations as evidence at a given time. There is active discussion about how to regulate learning algorithms in medicine – whether to treat each updated model as a new “device” requiring evaluation, or to allow autonomous learning under oversight guardrails.
7.2 Bias, Fairness, and Equity
AI systems are only as good as the data they are trained on. If the training data carries biases (which human-generated data often does), the AI can not only reflect but amplify biases. In healthcare, this has critical implications:
- Bias in Data: Historical healthcare data may reflect disparities. For example, if a minority group received less frequent treatment or had worse outcomes due to social factors, an AI predicting outcomes or making recommendations might incorrectly “learn” that being in that minority group inherently means a worse prognosis, and thus offer less aggressive treatment. This would perpetuate inequities. There have been documented cases of algorithmic bias – one notable example was an algorithm for guiding extra care programs that was less likely to refer black patients than white patients at the same level of illness, because it used healthcare costs as a proxy for health, and historical spending was lower for black patients despite similar needs.
- Ensuring Fairness: An ethical mandate for AI in EBM is to detect and correct such biases. Strategies include using bias mitigation in model training, such as re-weighting data or including fairness constraints so that the model’s recommendations do not discriminate against protected groups. It also includes validating AI tools separately in different demographic and socioeconomic groups to ensure they perform equally well. For example, an AI dermatology diagnostic tool trained mostly on images of lighter skin might fail on darker skin if not addressed; awareness of this led researchers to now ensure diverse training sets for such tools.
- Transparency about Bias: Ethical practice may require that if an AI tool’s performance varies by group, that should be known and taken into account. If an algorithm for predicting genetic risk was built mostly on European ancestry genomes, a clinician should know that its accuracy for non-European ancestry patients is lower, and thus not lean on it too heavily or seek a better-suited tool. From an EBM perspective, that’s like knowing the limits of a study’s generalizability.
- Potential to Reduce Bias: Interestingly, AI also has the potential to reduce human biases. Humans can be influenced by stereotypes or fallacies; a well-designed AI focuses on data and might ignore irrelevant factors like patient demographics except where truly medically relevant. For instance, clinical decision support that reminds the same evidence-based steps for everyone could standardize care and reduce variability due to individual provider biases. However, this ideal holds only if the AI is carefully checked for bias itself.
The ethical principle of justice in medicine demands that AI not create or worsen health disparities. This means extra caution and possibly regulatory oversight focusing on AI fairness. It might involve including ethicists and patient representatives in AI development and deployment discussions. In some cases, it might be ethical to purposely constrain an AI if it’s found to be using a variable in a way that is statistically sound but socially unjust – for example, an AI might predict slightly lower success for a treatment in an older patient and thus not recommend it, but if that difference is due to how older patients were managed historically (rather than true biology), we may decide ethically that age should not be used to deny treatment. This interplay of statistics and values is tricky and under active debate.
7.3 Accountability, Consent, and Trust
The introduction of AI into decision-making blurs lines of responsibility. If a physician follows an AI recommendation that leads to a bad outcome, who is accountable? Legally and ethically, currently the physician is – since AI tools are typically considered advisory. However, as AI becomes more integrated, this could create tension: physicians might be expected to use approved AI tools (failure to use them could be seen as negligent if they are proven to improve outcomes), yet if they use them and something goes wrong, the blame might still fall on the human. Manufacturers or developers of AI might bear some responsibility if their tool was flawed or used outside its intended setting.
Clear guidelines will be needed. Some suggestions:
- Physicians should understand the basics of any AI tool they use – its intended purpose, its limitations, and have the ability to override it. Essentially, AI should support, not replace, clinician judgment. If something doesn’t fit the clinical picture, the human must veto the AI. This is keeping with EBM: external evidence (even from AI) must be integrated with clinical expertise.
- Informed patient consent could evolve to include AI. Patients might need to be informed that AI is part of their care process, especially if it’s directly influencing decisions. Some ethicists argue patients have a right to know if, say, an algorithm helped decide their treatment plan, much as they have a right to know the risks and benefits. On the other hand, if AI is deeply embedded and always used, it might be considered part of standard of care not requiring special disclosure (we don’t usually explicitly tell patients “we used a calculator to figure your drug dose,” for instance). Where AI might have more direct patient interaction (like chatbot triage or AI in mental health apps), consent and transparency become even more important.
- Trust is a big issue. Patients trust clinicians in part because of the human relationship and the assumption the clinician has their best interest at heart (the ethical principle of beneficence). Will patients trust recommendations if they know “a computer” made them? It may depend on how well we can explain or validate those recommendations. Over time, if AI recommendations consistently lead to good outcomes, trust may build (much as autopilot is trusted in aviation). But any high-profile failures of medical AI could severely damage public trust. Therefore, a cautious and evidence-based rollout of AI (with pilot studies, publication of results, peer review, regulatory approval where appropriate) is essential to building confidence. Professional medical societies and regulators endorsing certain AI tools can also lend credibility.
- Autonomy of the patient remains paramount. There is a concern that AI might push a one-size-fits-all answer, potentially sidelining patient preferences. Clinicians must guard against this by ensuring the patient’s voice is still central. Ethically, a patient should not feel coerced by “the computer said so.” They should be part of the conversation about options and values. Ideally, AI could even facilitate patient autonomy by providing tailored information to patients (some envision AI personal health assistants that educate patients about their conditions and options in an accessible way, empowering them to engage in shared decision-making).
7.4 Ethical Practice in an AI-Enhanced EBM Environment
The use of AI in EBM doesn’t introduce wholly new ethical principles – it challenges us to apply existing ones (beneficence, non-maleficence, autonomy, justice) in new contexts:
- Beneficence and Non-Maleficence: We must ensure AI tools truly benefit patients (improving outcomes, experiences) and do not harm. That involves thorough testing, monitoring for errors or unsafe recommendations, and having fail-safes. An AI might inadvertently recommend something dangerous if a rare scenario wasn’t in training data – clinicians need to catch those. Some experts suggest having “sentinel” monitoring of AI decisions: e.g., random audits of AI-guided decisions to ensure they align with clinical sense and outcomes, at least in early adoption phases.
- Patient Autonomy: Even with an AI-proposed plan, patients have the right to accept or refuse. We need to communicate that AI suggestions are just that – suggestions, based on aggregated evidence, but the final choice lies with the patient (and clinician). If patients want a different route (within reason and safety), that should be respected. It’s akin to a patient choosing a non-standard but evidence-informed approach due to personal values.
- Privacy and Data Ethics: AI thrives on data, often needing large datasets, including patient records, to train. This raises privacy concerns. When using patient data to train AI (even in de-identified form), there are ethical duties to ensure it’s done with proper consent or at least under frameworks that protect patient rights (some jurisdictions treat de-identified data as outside consent requirements, others are more cautious). Also, data security is paramount – large datasets are targets for breaches. Ethical AI use means robust data protection measures.
- Intellectual Property and Access: Many AI tools are developed by private companies and may be proprietary. This can lead to unequal access – wealthy health systems might afford them, others not, potentially widening care gaps. An ethical standpoint would push for equitable access, maybe through open-source tools or inclusion of AI tools in public health packages. There is also the question of transparency vs. trade secret: some companies might not want to reveal how their AI works (for competitive reasons), but that clashes with the need for scrutiny in healthcare. Regulators might need to enforce at least some level of transparency or independent audit, even if the inner workings remain confidential publicly. The Vancouver (and broader scientific) ethos of reproducibility and openness runs into conflict with black-box commercial AI.
Lastly, the role of the physician may evolve ethically. With AI handling more analytical tasks, the physician’s relative role might shift towards more humanistic aspects – communication, empathy, understanding the patient’s life context. This could be a positive development: freeing doctors from some rote cognitive tasks to focus on caring and understanding. But it also requires that medical education adapt – tomorrow’s doctors need not only medical knowledge and EBM training, but also data literacy to work with AI and strong interpersonal skills to handle the parts AI can’t. Maintaining professional values (compassion, dedication to patients) is crucial so that the technology augments rather than diminishes the therapeutic relationship.
In conclusion, integrating AI into EBM carries significant epistemological and ethical implications that must be proactively managed. The promise is great – more precise, timely, and broadly informed care – but so are the pitfalls if not carefully guided. The marriage of AI and EBM will necessitate new standards for evaluating evidence, rigorous approaches to eliminate bias, clarity in roles and responsibilities, and unwavering commitment to patient-centered ethical care. Just as the pioneers of EBM brought a critical lens to medical tradition, the current generation must critically appraise AI and shape it to serve, not subvert, the foundational goals of medicine.
Chapter 8: Conclusion and Future Perspectives
Evidence-Based Medicine has traveled a remarkable journey: from its origins in the late 20th century as a radical shift toward “trust in numbers rather than tradition”, to its establishment as the bedrock of modern medical practice, and now into a future where those numbers are bigger and coming faster than ever before. This thesis has traced that journey through historical foundations, key transformations in practice and policy, and the exciting yet challenging frontier of artificial intelligence in healthcare.
In reviewing EBM’s historical foundations, we saw how visionary individuals like David Sackett and Iain Chalmers challenged the status quo of medical decision-making. Sackett’s work at McMaster University built the methodology and training grounds for EBM, emphasizing clinical epidemiology and critical appraisal. Chalmers, inspired by Archie Cochrane’s call to action, demonstrated the power of systematically reviewing evidence and led the creation of the Cochrane Collaboration in 1993, institutionalizing the practice of evidence synthesis. The rapid adoption of the EBM paradigm in the 1990s – with an explosion of EBM publications, journals, and textbooks – underscores how ripe medicine was for this change. In a sense, EBM answered a need that had become evident: the need for more reliable, transparent, and up-to-date information to guide patient care.
Through the principles and methodology of EBM, we highlighted how medicine became more of a science without losing its art. The requirement to integrate best research evidence with clinical expertise and patient preferences remains a guiding light. EBM’s tools – from the PICO question to the forest plot of a meta-analysis – have become part of the lexicon of healthcare. They have improved how we educate clinicians and how we systematically approach uncertainty. The evidence hierarchy and insistence on rigorous methods revolutionized clinical research itself, raising the bar for what counts as convincing evidence (e.g., the ubiquity of RCTs and meta-analyses in informing guidelines). EBM also laid bare where evidence was lacking, thus driving new research in areas of uncertainty.
The transformative impact on clinical practice has been profound. EBM has directly led to measurable improvements in outcomes by promoting interventions that work (e.g., timely reperfusion in heart attacks, use of antenatal steroids in preterm labor) and curbing those that don’t (e.g., unnecessary antibiotics, certain high-risk surgeries). It changed the physician’s approach from one of inherited habits to one of inquisitive skepticism – always asking “what is the evidence?” This cultural shift has arguably made medicine more humble and more dynamic; therapies are no longer used simply because “we’ve always done it” if evidence shows they are ineffective or harmful. Patients, too, are benefitting not only from better treatments but from being more involved in their care decisions, as clinicians share evidence with them and practice shared decision-making.
At the health system and policy level, EBM’s influence is seen in the proliferation of evidence-based guidelines, quality standards, and health technology assessments. Medicine has become more transparent and accountable – healthcare providers are expected to justify interventions with evidence, and payers/insurers increasingly demand that treatments be evidence-supported for reimbursement. While this can be contentious, it has generally steered resources towards interventions with proven value and away from wasteful spending on low-value care. EBM has also fostered international collaboration (e.g., through Cochrane and other networks) that harmonize and disseminate evidence globally, contributing to more equitable care standards across regions.
However, EBM as a movement has not been without critique and evolution. As noted, by the 2010s some felt EBM had drifted into a crisis of implementation – too much evidence to manage, potential overreliance on guidelines, and neglect of the individual patient in a sea of averages. The response from within EBM has been to emphasize “real EBM” – incorporating patient-centered care, acknowledging limitations of evidence, and focusing on meaningful outcomes. The advent of patient-oriented research, the GRADE system (which explicitly factors in patient values and context in recommendations), and the concept of minimally disruptive medicine for patients with multi-morbidity are all adjustments that refine EBM to be more fit for real-world practice.
Now, standing at the threshold of the AI era, EBM is poised to transform yet again. AI is not a replacement for the ethos of evidence-based medicine but a powerful set of tools to advance it. Properly harnessed, AI can help conquer the information overload that threatens to overwhelm clinicians, and it can surface insights from data that would otherwise remain hidden. We’ve explored how AI can make evidence synthesis faster and perhaps continuous, how it can personalize risk predictions and recommendations, and how it can serve as a safety net to ensure no relevant evidence is overlooked in patient care. These developments could lead to what has been termed “Evidence-Based Medicine 2.0”, where evidence is not static summaries published every few years, but a living, learning system integrated into care.
The future in the age of AI, however, demands vigilance to maintain the epistemological rigor and ethical compass of EBM. It would be all too easy to be seduced by complex algorithms and voluminous data and forget that at its core, medicine is about helping individual human beings. EBM taught us to question and verify – those skills will be just as important when evaluating AI recommendations or data-driven conclusions. Ensuring that AI interventions are themselves tested in robust trials or observational studies (analogous to the drug and device approval process) will be crucial before widespread adoption. In essence, we will need evidence-based evaluation of these new AI tools – a meta-level EBM.
Ethically, the medical community must guide AI development to prioritize patient welfare, equity, and respect for persons. This includes addressing biases in AI, being transparent about AI’s role in care, and educating both clinicians and patients so they understand the strengths and limits of AI contributions. Regulatory frameworks will need to evolve to provide oversight without stifling innovation – a challenging balance.
In a broader perspective, the fusion of AI and EBM could herald a new paradigm of “augmented medicine”. Clinicians augmented with AI might achieve outcomes previously unattainable, like curing diseases with highly individualized therapies or preventing illnesses through early prediction. But no matter how advanced decision-support becomes, the relational aspect of care – listening to patients, understanding their lives, providing compassion – will remain something that neither EBM’s evidence nor AI’s algorithms can replace. In fact, by offloading analytical burdens, clinicians might have more bandwidth for these human aspects, achieving the ideal that the science of medicine (the evidence) supports the art of medicine (the human touch).
As we conclude, one might ask: will evidence-based medicine even be a distinct concept in the future, or simply “medicine”? In many ways, EBM has become nearly synonymous with good practice. Perhaps in the future, as AI becomes seamlessly integrated, we won’t speak of “AI in medicine” either – it will just be part of medicine. The hope is that by then, through careful effort now, both evidence and AI will be so well woven into the fabric of healthcare that they quietly and efficiently enhance every consultation, every diagnosis, every treatment decision.
In summary, EBM has drastically improved the practice of medicine by grounding it in research and reason. Its ongoing evolution, especially in concert with AI, holds great promise for further improvements in effectiveness, efficiency, and equity of care. Yet, the core values must persist: a commitment to scientific inquiry, a dedication to patient-centered care, and an unwavering ethical grounding. The future of EBM in the age of AI will be shaped by how well we uphold these values while embracing innovation. If we succeed, the ultimate beneficiaries will be our patients, who will receive care that is not only cutting-edge and evidence-informed, but also compassionate and tailored to what matters most to them. This, truly, is the vision of evidence-based medicine fulfilled.
References
- Sackett DL, Rosenberg WM, Gray JA, Haynes RB, Richardson WS. Evidence based medicine: what it is and what it isn't. BMJ. 1996;312(7023):71-72.
- Guyatt GH, Cairns J, Churchill D, et al. Evidence-Based Medicine Working Group. Evidence-based medicine: A new approach to teaching the practice of medicine. JAMA. 1992;268(17):2420-2425.
- Cochrane AL. Effectiveness and Efficiency: Random Reflections on Health Services. London: Nuffield Provincial Hospitals Trust; 1972.
- Chalmers I. The Cochrane Collaboration: preparing, maintaining, and disseminating systematic reviews of the effects of health care. JAMA. 1995;274(24):1935-1938.
- Chalmers I, Enkin M, Keirse MJ, eds. Effective Care in Pregnancy and Childbirth. Oxford: Oxford University Press; 1989.
- Cochrane Collaboration. History of the Cochrane Collaboration. Cochrane Training Handbook 2023.
- Wennberg JE. Population illness rates do not explain population hospitalization rates. Health Aff (Millwood). 1987;6(1):39-51.
- Eddy DM. Practice policies: where do they come from? JAMA. 1990;263(9):1265-1275.
- Greenhalgh T, Howick J, Maskrey N. Evidence based medicine: a movement in crisis? BMJ. 2014;348:g3725.
- Bolt T, Huisman F. David Sackett and the emergence of clinical epidemiology: the confluence of personal ambition and social vision. J Clin Epidemiol. 2016;69:112-116.
- Haynes RB, Devereaux PJ, Guyatt GH. Clinical expertise in the era of evidence-based medicine and patient choice. ACP Journal Club. 2002;136(2):A11-A14.
- Grading of Recommendations Assessment, Development and Evaluation (GRADE) Working Group. GRADE Handbook. Updated 2013. Available from: https://gdt.gradepro.org/app/handbook/handbook.html.
- Institute of Medicine (IOM). Clinical Practice Guidelines We Can Trust. Washington, DC: National Academies Press; 2011.
- McGlynn EA, Asch SM, Adams J, et al. The quality of health care delivered to adults in the United States. N Engl J Med. 2003;348(26):2635-2645.
- Shelton JB, Saigal CS. The crossroads of evidence-based medicine and health policy: implications for urology. Int Braz J Urol. 2011;37(4):398-408.
- Topol EJ. High-performance medicine: the convergence of human and artificial intelligence. Nat Med. 2019;25(1):44-56.
- Nilsen P, et al. Towards evidence-based practice 2.0: leveraging artificial intelligence in healthcare. Front Digit Health. 2024; (In press).
- Liu X, Rivera SC, Moher D, et al. Reporting guidelines for clinical trial reports for interventions involving artificial intelligence: the CONSORT-AI extension. Nat Med. 2020;26(9):1364-1374.
- Coiera E. The fate of medicine in the time of AI. Lancet. 2018;392(10162):2331-2332.
- Esteva A, Robicquet A, Ramsundar B, et al. A guide to deep learning in healthcare. Nat Med. 2019;25(1):24-29.
- Finlayson SG, Subbaswamy A, Singh K, et al. The clinician and dataset shift in artificial intelligence. N Engl J Med. 2021;385(3):283-286.
AI Reasoning
Evidence-Based Medicine: Historical Foundations, Transformative Impact, and Future in the Age of AI
I’m piecing together AI in EBM, covering predictive modeling, systematic reviews, decision support, and ethical implications. Progress is steady through research and analysis.
Searched for David Sackett evidence-based medicineChatGPT
I'm piecing together the importance of result 0 and 1, leaning towards credible sources like journal articles.
ChatGPT
Sackett's death in 2015, exactly 25 years after EBM was coined around 1990, underscores the shift from trust in experts to trust in data.
Read blog.library.gsu.edu
ChatGPT
Sackett's pioneering roles in Clinical Epidemiology and the Oxford Centre for EBM shaped EBM, emphasizing the marriage of expertise with systematic research evidence.
Read more from blog.library.gsu.edu
ChatGPT
OK, let's clarify the definition of Evidence-Based Medicine (EBM) as outlined by Sackett et al., emphasizing integrating clinical expertise with external research evidence.
Searched for Iain Chalmers Cochrane Collaboration historyChatGPT
I’m evaluating a BMJ article and Cochrane's site for Iain Chalmers' context, considering "Chapter I: Introduction - Cochrane Training."
ChatGPT
I’m mapping out the origins of EBM. Archie Cochrane's 1979 vision catalyzes Iain Chalmers and colleagues at McMaster, leading to the Cochrane Collaboration.
Read evidenceprime.com
ChatGPT
AI's role in evidence-based medicine includes detecting research gaps, accelerating evidence synthesis, and enhancing patient engagement and coordinated care, presenting promising solutions for many current challenges.
Searched for machine learning in Cochrane reviews automation
ChatGPT
The Cochrane resource focuses on training and implies AI exploration. This chapter should cover AI in detecting research gaps, speeding reviews, incorporating patient values, and predictive modeling, emphasizing its impact on evidence-based medicine.
Searched for AI in evidence-based medicine predictive modeling
Read pmc.ncbi.nlm.nih.gov
ChatGPT
Listing issues like slow evidence generation, applicability differences, and cognitive biases.
AI assists with evidence generation, synthesis, and clinical practice integration, showing promise especially in image analysis.
"EBP 2.0" concept is introduced, highlighting the uncertain future and need for further research to validate its efficacy.
Read more from pmc.ncbi.nlm.nih.gov
ChatGPT
EBM, championed by the McMaster EBM Working Group in the early 90s, expanded integration with tech and spread to fields beyond medicine, overcoming barriers and adapting to critiques.
Searched for Greenhalgh EBM movement crisis 2014ChatGPT
Trisha Greenhalgh's 2014 piece critiques evidence-based medicine (EBM) for excessive volume, industry influence, and guideline rigidity, proposing patient focus to renew EBM.
Read bmj.com
Read s4be.cochrane.org
ChatGPT
Summarizing main critiques of evidence-based medicine (EBM)—industry bias, overwhelming evidence volume, limited trial outcomes, rigid doctor adherence, and lack of adaptability. EBM's evolution involves historical figures and their roles.
Searched for Gordon Guyatt evidence-based medicine term originChatGPT
Created by McMaster's Program Director Gordon Guyatt in 1990, likely published in 1991 ACP J Club editorial.
Read journalofethics.ama-assn.org
ChatGPT
I am mapping out the emergence of "evidence-based medicine" in the 1990s, focusing on Gordon Guyatt, David Sackett, and Iain Chalmers, and the influence of Archie Cochrane's critiques.
Read bmj.com
Searched for Iain Chalmers Cochrane biography
Read en.wikipedia.org
ChatGPT
Organizing the chapters and citations involves detailing historical foundations, AI applications, and ethical concerns, while adhering to Vancouver style references.
Read thelancet.com
ChatGPT
I'm thinking through the resources we have, including references on the impact of Evidence-Based Medicine (EBM) on health policy, and considering Wikipedia for additional information.
Searched for evidence-based medicine impact on health policyChatGPT
Analyzing how EBM and policies intersect, I delve into their integration and implications in this new section.
See how EBM is shaping policy, aiming to cut costs by removing unnecessary evidence-based care and boosting quality. Discussing its impact on reforms like the Affordable Care Act.
Read pmc.ncbi.nlm.nih.gov
ChatGPT
Tracing the evolution of evidence-based medicine, concluding with McGlynn et al's 2003 study showing 55% compliance with guidelines.
Read more from pmc.ncbi.nlm.nih.gov
ChatGPT
Tracing the "evidence-based" term's origin with David Eddy, examining practice variation by Wennberg. Addressing guideline gaps, noting EBM's influence on quality measures and AI implications.
Searched for AI in evidence-based medicine ethical implicationsChatGPT
I'm thinking through trust in AI, the nature of evidence, and the need for validation by EBM methods or RCTs.
Searched for epistemology in evidence-based medicine AIChatGPT
I'm pulling together ideas on machine learning's impact on Evidence-Based Medicine (EBM). Assessing if the full article is accessible without a paywall.
Read sciencedirect.com
ChatGPT
Piecing together sources from BMJ, JAMA, and Cochrane's work to manually craft a comprehensive references list.
ChatGPT
I’m thinking through whether [19] refers to Archie Cochrane's lecture or an anniversary. Considering Cochrane's foundational work from 1972 or Iain Chalmers' early papers on the Cochrane Collaboration.
Searched for Chalmers 1995 BMJ Cochrane reviewsChatGPT
I'm gathering info on the JAMA 1995 article by Chalmers about the Cochrane Collaboration. Also considering a 1996 BMJ article by Sackett for EBM principles.
Read ncbi.nlm.nih.gov
ChatGPT
I’m gathering key references for the thesis, including foundational works on evidence-based medicine and AI in healthcare.
Read blog.library.gsu.edu
ChatGPT
OK, let me see: Sackett pioneered the Clinical Epi dept at McMaster in 1967, co-editing "Evidence-Based Medicine," and chaired Cochrane Collaboration. Hm, that's interesting.
Read journalofethics.ama-assn.org
ChatGPT
I'm considering if the small image showing the rise in EBM papers should be included. It's historically interesting but may be too small to effectively visualize the growth.
Searched for evidence-based medicine hierarchy pyramid image
Searched for AI in evidence-based medicine decision support
Read evidenceprime.com
Read pmc.ncbi.nlm.nih.gov
Read s4be.cochrane.org
ChatGPT
It appears the image should be at page 53.
ChatGPT
I’m exploring AI’s role in evidence-based medicine for predictive modeling and decision support. It seems crucial for handling complexity and updating evidence swiftly.
I’m piecing together the role of AI in evidence-based medicine (EBM), considering its ethical integration to maintain humanistic care while reducing biases. Progress is being made towards a balanced approach.
ChatGPT
I’m pulling in Cochrane's quote to enhance the argument, making it more compelling and well-supported.
Read pmc.ncbi.nlm.nih.gov
ChatGPT
"I'm piecing together balanced sections on David Sackett's contributions to McMaster and Oxford, and Iain Chalmers' impact with the Cochrane Collaboration, ensuring a deep dive into each focus area."
Read more from pmc.ncbi.nlm.nih.gov
ChatGPT
I’m beginning the final content analysis, focusing on refining it with citations for clarity.
Read more from pmc.ncbi.nlm.nih.gov
ChatGPT
I’m integrating citations, images, and adhering to formatting guidelines to ensure the final answer is comprehensive and well-structured.